The rapid development of in vivo mesoscale imaging technology has made it possible to dynamically observe large-scale neural activity. High-precision data from large-scale neural recordings make the differences between repeated trials impossible to ignore. Traditional neuroscience has viewed these differences as unwanted noise and typically reduces their impact by averaging across multiple trials. While this approach can mitigate noise from biological and observational systems, it may also lead to the loss of critical information, especially in areas of the brain related to higher cognitive functions. After all, the brain does not understand the world through averaging; even when receiving the same repeated stimuli, its output often results in varied cognitive experiences. To further understand the mechanisms behind trial variability at the single-cell level across different scales, mesoscale whole-cortex observation technology becomes crucial.
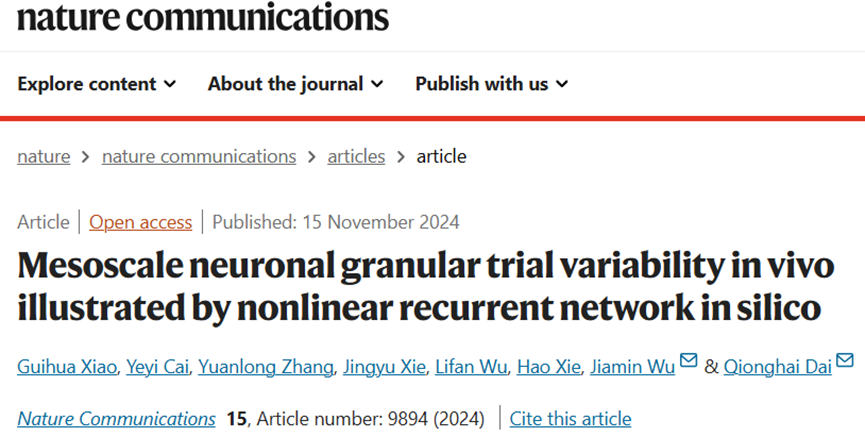
To understand and address the challenge of trial variability at the single-cell level, on November 15, 2024, Beijing time, the team of Qionghai Dai and Jiamin Wu from Tsinghua University published a paper titled "Mesoscale Neuronal Granular Trial Variability in Vivo Illustrated by Nonlinear Recurrent Network in Silico" in Nature Communications. The paper first hypothesizes that trial variability, whether in the brains of live animals or in computational models, is not merely noise but could be an intrinsic representation of neural activity. Based on the first-generation RUSH imaging system developed by their team in 2019, the paper proposes a novel mouse experimental paradigm that integrates mesoscale imaging and deep brain stimulation of the claustrum to achieve synchronization control. Under repeated electrical stimulation and varying stimulus intensities, dynamic neuronal activity responses across the entire cortex were recorded. The study documented the dynamic activity of nearly 40,000 individual neurons across 12 bilateral cortical regions. The authors established paradigms for mesoscale single-cell neural activity extraction, multimodal behavioral analysis, and synchronized stimulation analysis. The dynamic changes in neural activity in vivo demonstrated that trial variability is an inevitable result of the brain's dynamic information processing, influenced by the intensity and spread of external stimulation. Moreover, the paper developed a nonlinear recurrent neural network model, which confirmed the mechanism of trial variability through computational simulations. Despite the existence of trial variability at the single-cell level, the brain can still decode external stimuli through heterogeneous cortical activity, potentially forming the foundation of the brain's flexible readout strategies that support stable sensory representations.
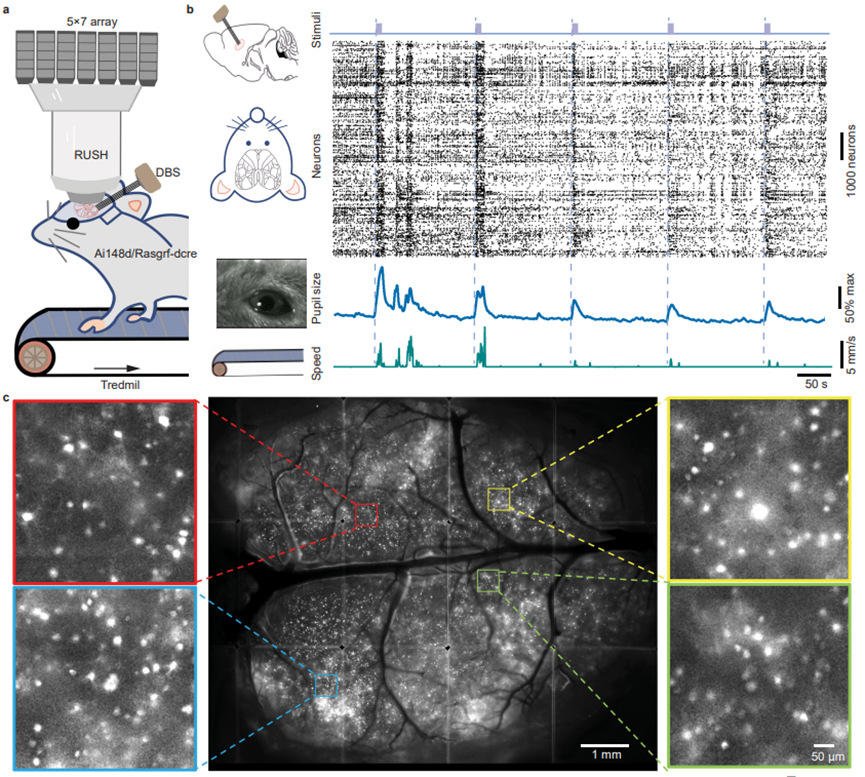
Figure 1. Paradigm for mesoscale whole-cortex neuronal activity and behavioral recording.
The study first used transgenic mice specifically expressing GCaMP6f in cortical layer 2/3 (Ai148d/Rasgrf-dcre). Using a carefully designed implantation strategy, a multi-channel electrical stimulation electrode was implanted into the deep brain region of the claustrum, while the entire cortex was exposed through craniotomy. After postoperative recovery, the mice were subjected to mesoscale neural activity observation under electrical stimulation control. Multimodal behavioral data and single-cell activity across the entire cortex were recorded and analyzed.
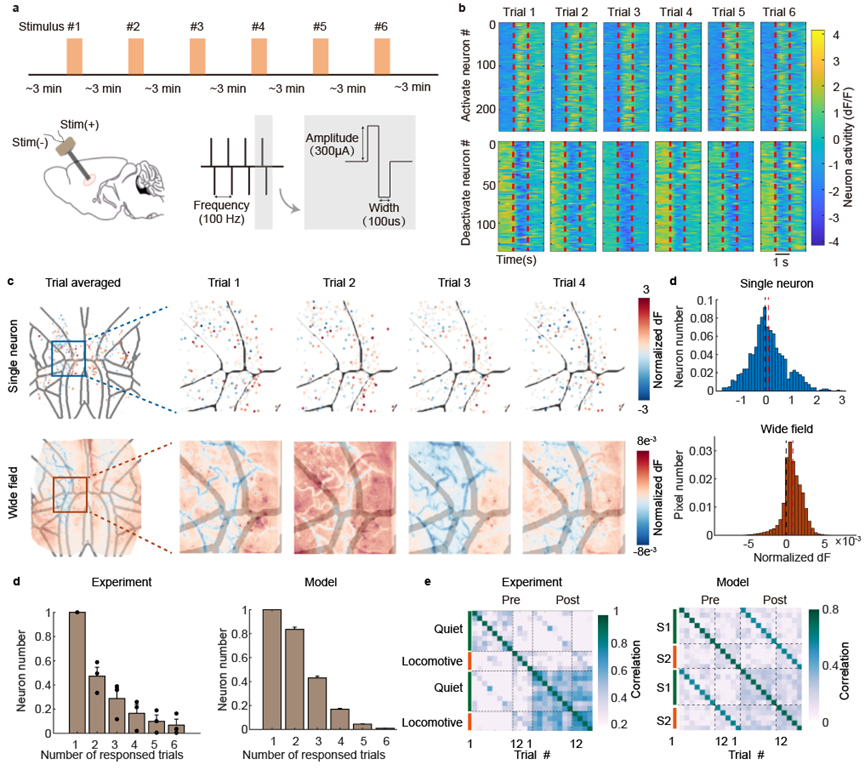
Figure 2. Changes in mesoscale neuronal activity under repeated electrical stimulation.
The study established a paradigm for cortical-wide neural activity observation under repeated electrical stimulation. Deep brain nucleus electrical stimulation is considered a passive input, which effectively minimizes variables that could affect trial variability, such as the animal's attention state, task engagement, arousal level, or eye movements. Electrical stimulation also allows for more flexible parameter control over stimulus intensity. During repeated stimulation with identical parameters, most neurons showed activation or inhibition. Trial variability was inevitably observed in both single-cell and regional neural responses, and it had a more pronounced impact at the single-cell level. From the nonlinear recurrent neural network model we constructed, similar results were seen, as observed in the living brain. Most neurons only responded to a single trial, and the responses were influenced by the animal's pre-stimulation motion state.
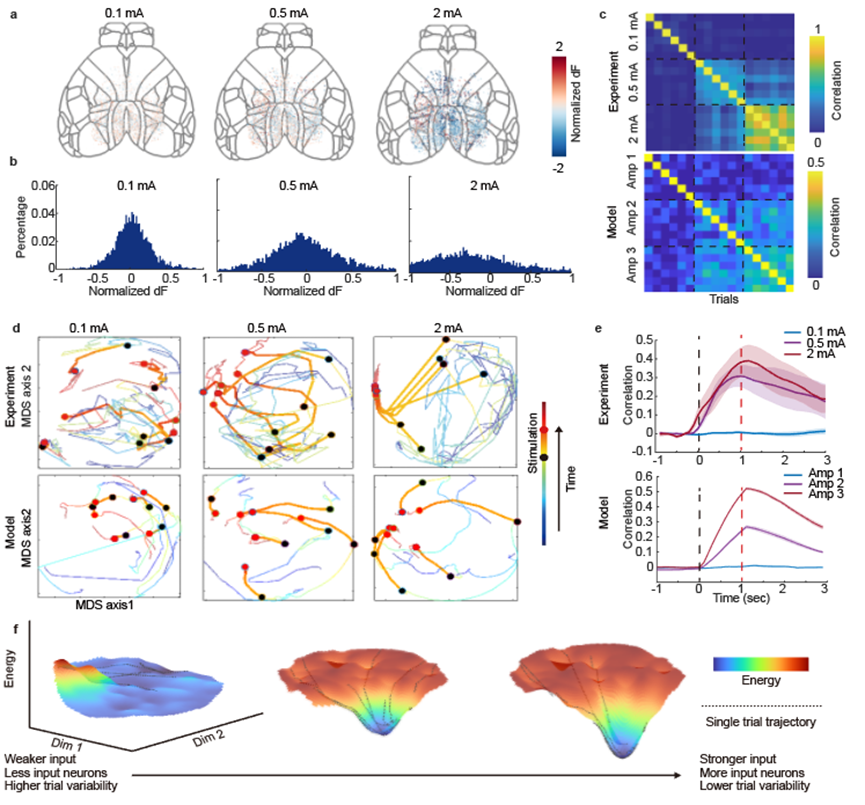
Figure 3. Changes in mesoscale neuronal activity under different amplitudes of electrical stimulation.
In the design of the computational model, two parameters are particularly critical: the amplitude of external stimulation and the size of the input neuron population. In the in vivo experiments described, the amplitude of electrical stimulation was modulated while keeping the stimulation energy within a tolerable range for the mice. As expected, larger input current amplitudes tuned more neurons in the cortex. Trial variability was quantified by the correlation among neuronal populations, and higher input currents led to increased correlation between trials, consistent with observations from the model. Visualized energy landscapes showed that stronger input and greater involvement of neurons in the task resulted in the brain state forming a more pronounced attractor in a shorter time.
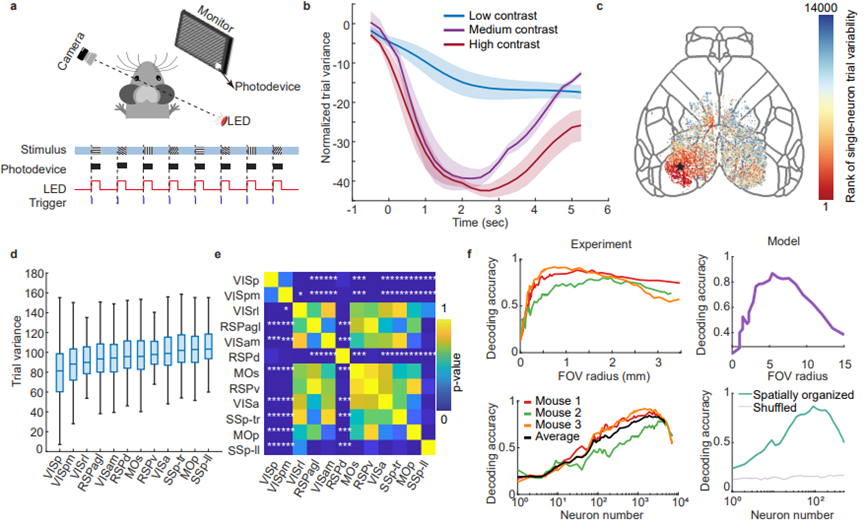
Figure 4. Mesoscale neuronal activity encoding under visual stimulation.
To further verify whether the brain follows the same pattern of trial variability under visual sensory stimulation, the study designed eight different directions of visual grating stimuli and varying contrast intensities. It was found that different sensory intensities of visual contrast follow similar patterns. As the stimulus intensity increased, trial variability between repeated trials decreased. This single-cell level variability displayed distinct distribution characteristics across different brain regions. While a large number of low-variability neurons were present in the primary visual cortex, other brain regions still showed a certain degree of neuron variability. To better understand the impact of such trial variability on the brain, the study directly used neuronal decoding accuracy as a measure of trial variability, which is crucial for applications like brain-computer interfaces that have limited neural recording channels. High decoding accuracy typically indicates low trial variability at the single-neuron or neuronal group level. The study calculated decoding accuracy using neurons within a specific radius centered in the visual cortex. As the field of view extended beyond the visual cortex, decoding accuracy initially increased and then steadily declined, suggesting that, beyond the visual cortex, noise contributions outweighed the information contributions. In simple tasks, expanding the field of view may not lead to better decoding performance.
Guahua Xiao, an Assistant Researcher at the National Research Center of Tsinghua University, and Yeyi Cai, a Ph.D. student from the Department of Automation, are the co-first authors of this paper. Professors Qionghai Dai and Jiamin Wu from Tsinghua University are co-corresponding authors, while Yuanlong Zhang, Jingyu Xie, Lifan Wu, and Hao Xie from the Department of Automation made significant contributions to this work.
Original article link:https://www.nature.com/articles/s41467-024-54346-3
Code link for the paper:https://github.com/Cai-yy/Single-trial-variability.git.