Microscopic images with high signal-to-noise ratios and high resolution consistently contain richer and more accurate information, helping us perceive the microscopic world with greater precision. However, traditional super-resolution (SR) microscopy techniques often sacrifice other important performance metrics, such as imaging duration and speed, while improving spatial resolution, due to limitations imposed by various biophysical, biochemical, and physical optical factors (such as fluorophore concentration, probe brightness, phototoxicity, photobleaching, and photon noise). In response to this issue, the collaborative team of Qionghai Dai and Dong Li at Tsinghua University proposed the Deep Fourier Channel Attention Network (DFCAN) in 2021, which can achieve high-fidelity SR predictions using only a single low-resolution image. Since then, several single image SR (SISR) models have been developed to enhance the capabilities of live-cell SR microscopy. However, these SISR models often encounter two major limitations when applied to actual live-cell microscopy experiments (typically represented as time-lapse data). Firstly, SISR models cannot capture the temporal dependencies between adjacent frames, thus yielding temporally inconsistent inferences. Secondly, existing SISR methods lack accurate evaluation on output confidence, making it hard for biologists to determine the reliability of the generated results.
To address the aforementioned challenges, on January 29, an academic paper titled “A neural network for long-term SR imaging of live cells with reliable confidence quantification” was published in the journal Nature Biotechnology by the team of Academician Qionghai Dai and Associate Professor Qiao Hui from the Department of Automation at Tsinghua University, in collaboration with Professor Dong Li's team from the School of Life Sciences at Tsinghua University. The team utilized their home-built Multimodality Structured Illumination Microscopy to construct a high-quality public dataset of time-lapse SR microscopy images, named BioTISR. Based on a systematic evaluation of existing time-lapse image SR (TISR) methods, they devised a deformable phase-space alignment (DPA) mechanism along with the corresponding TISR model (DPA-TISR). This model is capable of high-fidelity SR reconstruction of low-SNR, low-resolution time-lapse images, expanding the multi-color live SR imaging time series by over 30 times and extending it to over ten thousand time points. Furthermore, the team combined Bayesian learning with DPA-TISR to propose the Bayesian DPA-TISR, and developed a method to minimize the expected calibration error (ECE) for accurate confidence assessment of the DPA-TISR outputs, thereby assisting biologists in conducting more reliable quantitative research.
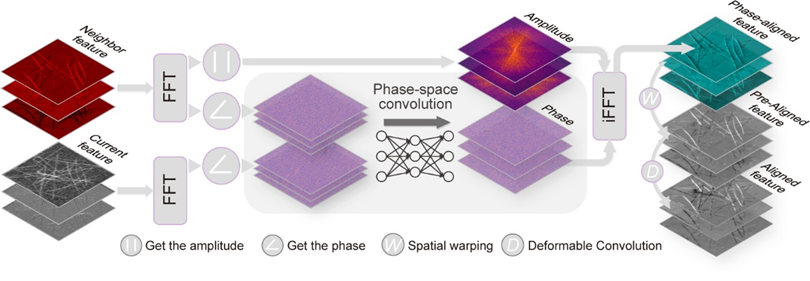
Figure 1. Schematic of the DPA mechanism
The research team first constructed a large-scale high-quality dataset for the biological time-lapse image SR task, named BioTISR, and utilized this dataset to conduct a comprehensive and systematic evaluation of two key components of TISR methods: the propagation mechanism and the alignment mechanism. The evaluation results indicated that even the most advanced deformable convolution alignment mechanism in existing methods failed to adequately capture the global, large displacements of biological structures between adjacent frames, resulting in suboptimal overall SR reconstruction. To address this issue, the team designed a time-lapse image SR neural network model called DPA-TISR, which is based on phase-space alignment (as illustrated in Figure 1). This model can adaptively enhance cross-frame alignment in the phase domain and achieves the current state-of-the-art performance in TISR. Together, the BioTISR dataset and the DPA-TISR model establish a benchmark and baseline for time-lapse microscopy image SR in the field of SR microscopy imaging. This advancement will further promote the deep integration and cross-innovation between the AI community and the optical microscopy imaging field.
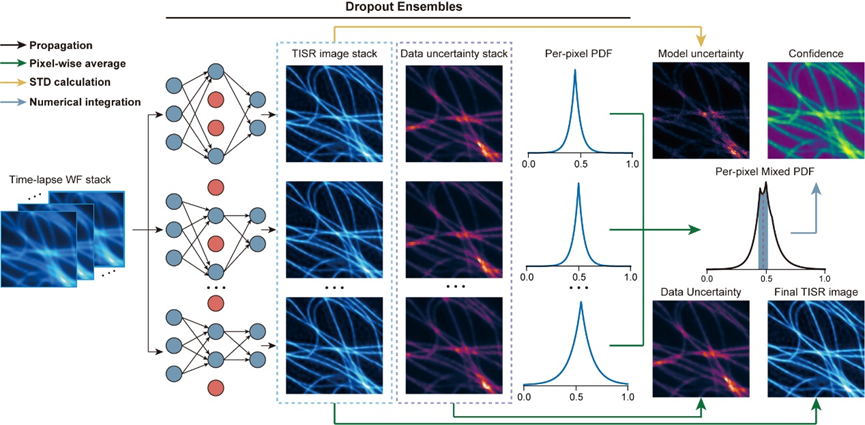
Figure 2. Bayesian Neural Network for Time-lapse Image SR.
Building on the DPA-TISR model, the research team addressed the challenges of uncertainty and unknown confidence levels in image SR neural networks by introducing Bayesian deep learning and a Monte Carlo dropout approach, resulting in the proposed Bayesian DPA-TISR (as shown in Figure 2). To tackle the common issue of overconfidence in Bayesian learning, the team designed an expected calibration error minimization method. Through iterative linear search and parabola fitting, they fine-tuned the neural network to ensure that the predicted confidence maps closely align with the actual errors. This approach successfully reduced the model's expected calibration error by more than five times, providing a reliable indicator of potential inference errors in the model.
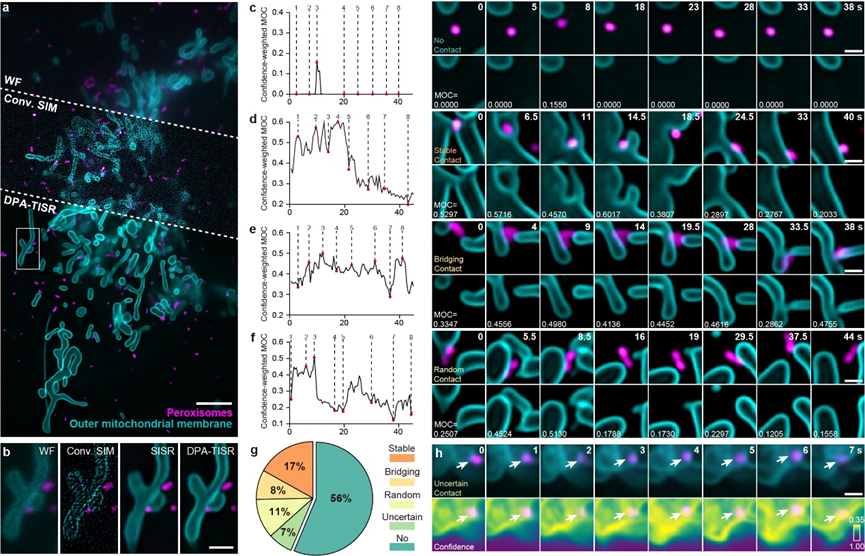
Figure 3. Classification and Evaluation of Mitochondrial and Peroxisomal Interactions.
The accurate confidence quantification capability of Bayesian DPA-TISR can significantly enhance rigorous life science research. For instance, peroxisomes (POs) are functional organelles that frequently interact with mitochondria to regulate cellular reactive oxygen species metabolism. However, due to limitations in observation techniques, the types and proportions of mitochondrial-peroxisomal interactions have not been systematically studied. Leveraging the high-speed, long-duration, and SR imaging capabilities of DPA-TISR, the research team was able to clearly distinguish the spatial relationships and interaction mechanisms between these two organelles (as shown in Figure 3). They discovered that nearly half of the POs never made contact with mitochondria, while the other half that did interact with mitochondria could be categorized into three types: 17% of POs stably associated with contact sites from a single mitochondrion; 8% of POs served as the bridge that simultaneously tethered two or more mitochondria; and 11% of POs unexpectedly changed their contact sites from one mitochondrion to another, acting as intracellular messengers. Notably, the final 7% of POs were classified as unidentified because Bayesian DPA-TISR indicated that the confidence level in the image for that region was too low to allow for reliable behavioral classification (as shown in Figure 3h). This study demonstrates that Bayesian DPA-TISR can provide a novel technical pathway and a broad application foundation for ultra-long-term live-cell SR imaging and confidence-quantifiable biological analysis.
Postdoctoral researcher Chang Qiao, doctoral students Shuran Liu, Wencong Xu from the Department of Automation at Tsinghua University, and Associate Researcher Yuwang Wang from the National Research Center for Information Science and Technology at Tsinghua University are the co-first authors of this article. Professor Qionghai Dai and Associate Professor Hui Qiao from the Department of Automation, the National Research Center for Information Science and Technology, and the Institute for Brain and Cognitive Sciences at Tsinghua University, along with Professor Dong Li from the School of Life Sciences at Tsinghua University, are the corresponding authors of this paper. The research was supported by the National Natural Science Foundation of China, the National Key Research and Development Program of China, the Beijing Natural Science Foundation, the Tencent New Cornerstone Science Foundation, the Shui Mu Tsinghua Scholar Program, and the China Postdoctoral Science Foundation.
[1] Qiao, C. et al. Evaluation and development of deep neural networks for image super-resolution in optical microscopy. Nat. Methods 18, 194–202 (2021).
Original article link:https://www.nature.com/articles/s41587-025-02553-8