The study of the interactions and functions among multiple cells and organelles within biological organisms relies heavily on the continuous advancement of live imaging techniques, especially those with high spatiotemporal resolution to capture rapid three-dimensional changes in the complex microenvironments inside mammalian bodies. Over the past decade, scientists have made significant efforts in innovating three-dimensional fluorescence imaging techniques, facilitating rapid advancements in disciplines such as cell biology, developmental biology, and neuroscience. Among these innovations, the scanning light field microscope (sLFM) proposed by the Imaging and Intelligent Technology Laboratory of Tsinghua University stands out as a typical representative of computational microscopy. Leveraging its high-speed three-dimensional imaging capability, digital adaptive optics, and low phototoxicity, sLFM has been widely employed in studies of oncology, immunology, neuroscience, and other fields across various animal models. Although existing physical scanning processes have overcome the contradiction between spatial resolution and angular resolution in light field imaging, they have also led to issues such as reconstruction artifacts and resolution degradation when capturing ultra-high-speed biological phenomena, such as cells in rapid blood flow and neuronal voltage responses. These limitations restrict the spatiotemporal resolution of current live three-dimensional microscopic imaging.
In response to this challenge, on April 6, 2023, the research paper titled "Virtual-scanning light-field microscopy for robust snapshot high-resolution volumetric imaging" by the team led by Prof. Qionghai Dai from the Imaging and Intelligent Technology Laboratory at Tsinghua University was published online in Nature Methods. The team, building upon scanning light field microscopy, released the world's first large-scale, multi-sample microscopic light field super-resolution dataset. They further proposed the Virtual-scanning Light Field Microscopy (VsLFM) technique, which, in conjunction with scanning light field systems, enables the restoration of single-exposure light field microscopy images to three-dimensional diffraction-limited resolution on approximate sample structure data. This achievement marks the first-ever 500Hz three-dimensional subcellular resolution voltage imaging of the entire fruit fly brain range. [2]。
VsLFM exploits the physical constraints of frequency aliasing in multi-angle views caused by microlens aperture diffraction. It constructs a physics-informed deep neural network, Vs-Net, through large-scale datasets and deep learning to address the signal processing challenge of frequency aliasing. Compared to previous deep learning methods, VsLFM significantly enhances interpretability and generalization. Its openly available pre-trained models can be widely applied to different sample structures, enabling the process of virtual digital scanning to replace physical scanning. This approach exhibits ultra-high spatiotemporal resolution under single-exposure conditions while effectively removing motion artifacts. It is better suited for imaging within natural rhythms such as heartbeat, respiration, and blood flow in animal bodies, thereby comprehensively expanding the application scope and system robustness of scanning light field microscopy.
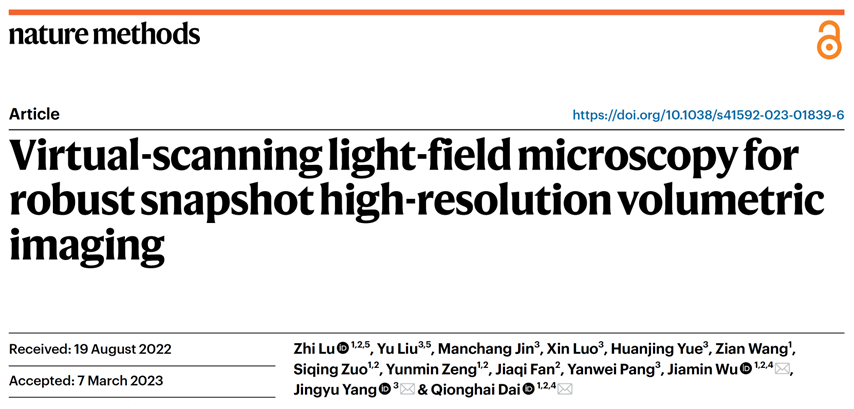
The core principle of VsLFM involves an organic fusion of the dimensionality correlation of light field images with the strong fitting capability of deep neural networks. As illustrated in Figure 1, Vs-Net fully utilizes the physical priors of phase correlation between different angles introduced by microlens diffraction (a factor typically ignored in macroscopic light field image processing). By simultaneously considering multiple angular measurement images during the upsampling process, Vs-Net extracts high-dimensional features designed for spatial-angular, light field, and angular aliasing, recovering high-frequency structural information from complex spatial-angular frequency aliasing. Following Vs-Net, the point spread function (PSF) in the light field phase space, acting as another physical prior, facilitates the three-dimensional reconstruction of high-resolution light field images, preserving the capability for digital adaptive optics aberration correction, and achieving diffraction-limited resolution.
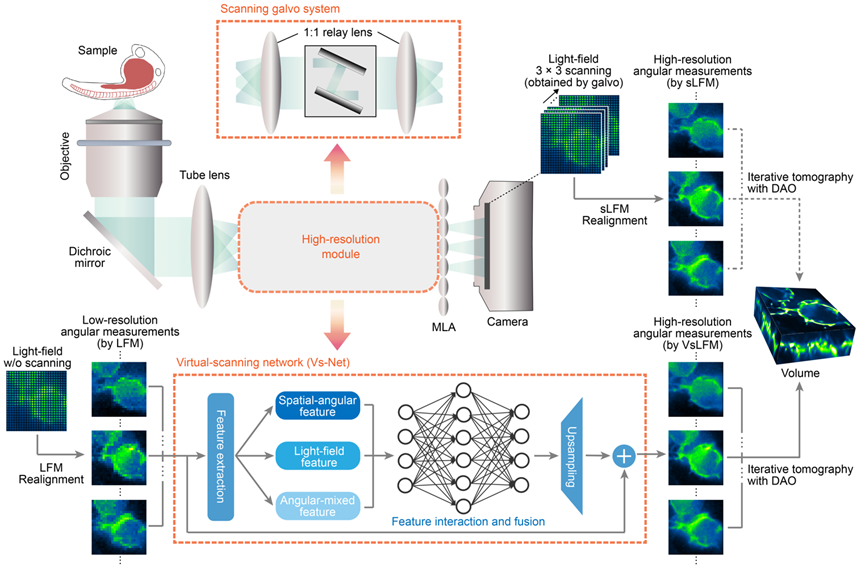
Figure 1. Principle of VsLFM
Traditional LFM and end-to-end LFM reconstruction neural networks have long been unable to achieve subcellular resolution within micrometers or exhibit low generalization due to the ill-posed nature of the inverse problem in the reconstruction process, making practical applications difficult. VsLFM, a physics-informed distributed learning framework, addresses this challenge by introducing phase correlation between multiple viewpoints and the wave-like diffraction of the point spread function, bridging the huge resolution gap of over 10 times between light field images and three-dimensional volumes. As shown in Figure 2, researchers conducted tests on WGA-labeled L929 cells, comparing the performance of different deep learning methods trained on online mitochondrial data and tested on actin data. Compared to previous methods, VsLFM demonstrates the highest spatial resolution and robustness, possesses sufficient generalization capabilities, and avoids network reconstruction artifacts.
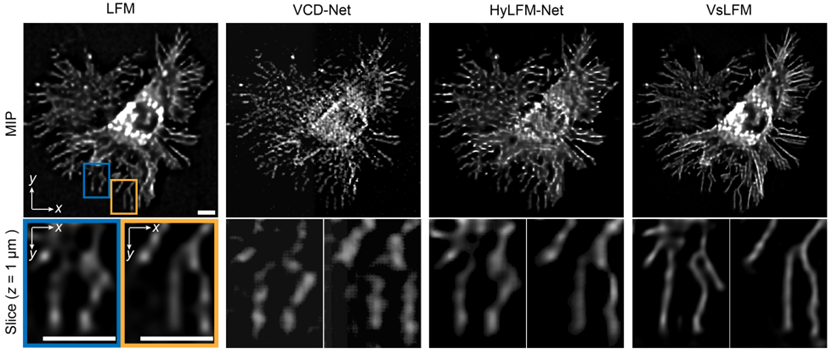
Figure 2. High resolution and strong generalizability of VsLFM
Unlike ex-vivo imaging, in vivo imaging is often accompanied by significant photon noise and optical aberrations due to tissue heterogeneity and photosensitivity. This poses a significant challenge for computational microscopy techniques that rely on accurate imaging models. Due to limitations in datasets, end-to-end network training is typically restricted to specific imaging conditions, whereas VsLFM, with its distributed strategy and physics-constrained priors, can maintain high imaging performance in complex scenarios. To assess this robustness, researchers first compared the imaging performance of VsLFM with two end-to-end networks in noisy scenarios. These networks were trained under high signal-to-noise ratio conditions and tested under photon-limited conditions. As shown in Figures 3a-3c, pre-trained end-to-end networks have strict imaging condition requirements, leading to potential overfitting, signal-to-noise confusion, and severe artifacts and structural fragmentation. In contrast, VsLFM, leveraging physical constraints between angular views, effectively distinguishes signals from strong noise, resulting in a significant improvement in fidelity under low-light conditions. On the other hand, tissue heterogeneity introduces significant optical aberrations, disrupting the mapping relationship learned by end-to-end models and causing visible distortions and artifacts. VsLFM employs digital adaptive optics for distortion correction, achieving superior image quality, as shown in Figures 3d-3e.
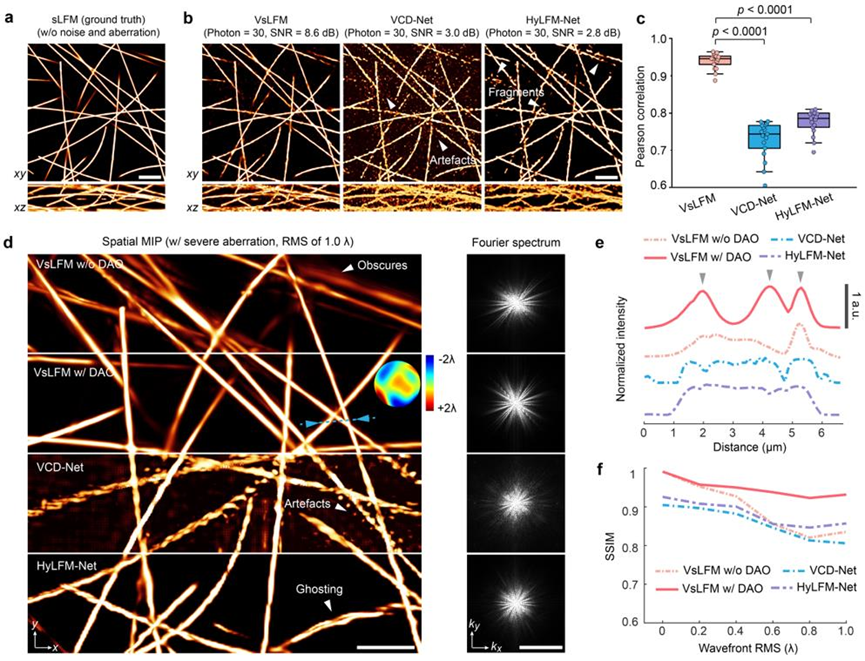
Figure 3. VsLFM demonstrates robustness in complex scenarios involving noise, aberrations, and other factors.
With its single-exposure near-diffraction-limited three-dimensional imaging capability and robustness in complex scenes, VsLFM fills the gap in analyzing subcellular dynamic changes in high-speed and complex environments within mammals. Researchers applied VsLFM to monitor immune cells in the liver of live mice. As shown in Figure 4, strong motion such as blood flow within the mouse body inevitably disrupts the physical scanning mode during sLFM acquisition, resulting in motion artifacts and low temporal resolution. However, VsLFM effectively eliminates severe motion artifacts, maintaining the intact shape of neutrophils, and combining the high speed of LFM with the high resolution of sLFM.
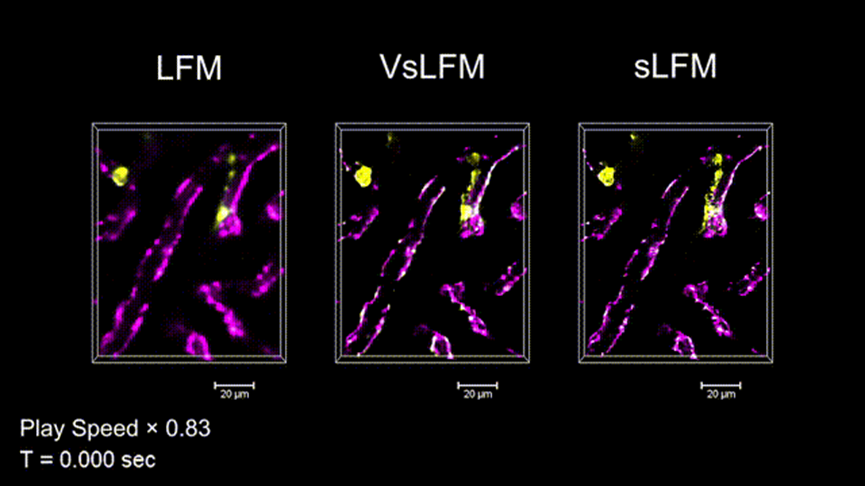
Figure 4. VsLFM effectively eliminates motion artifacts from rapidly moving cells while preserving high resolution (GIF).
Based on this powerful capability, researchers further attempted to observe ultra-high-speed 3D voltage activity. Live voltage recording is crucial for studying the complex interactions between learning units in the animal nervous system and the feedback interconnections between short-term and long-term memory. However, achieving dynamic capture of subcellular resolution three-dimensional voltage signals over a large range has been elusive due to the extremely fast three-dimensional voltage transients exceeding 200Hz and the low signal-to-noise ratio resulting from short exposure times. As shown in Figure 5, leveraging VsLFM, researchers have, for the first time, achieved sub-micrometer resolution and 500Hz ultra-high spatiotemporal resolution in recording the 3D voltage activity of sparse dopamine neurons in the entire Drosophila brain. They observed the three-dimensional propagation of action potentials in axonal branches and dendritic arbors of PL1-α'2α2 neurons within a 2.4-millisecond time window. Additionally, they validated the elevation of voltage activity firing rates during odor stimulation in vivo. With its ultra-high spatiotemporal resolution and strong robustness, VsLFM holds promise for conducting more extensive studies on complex neural activities in various animal models.
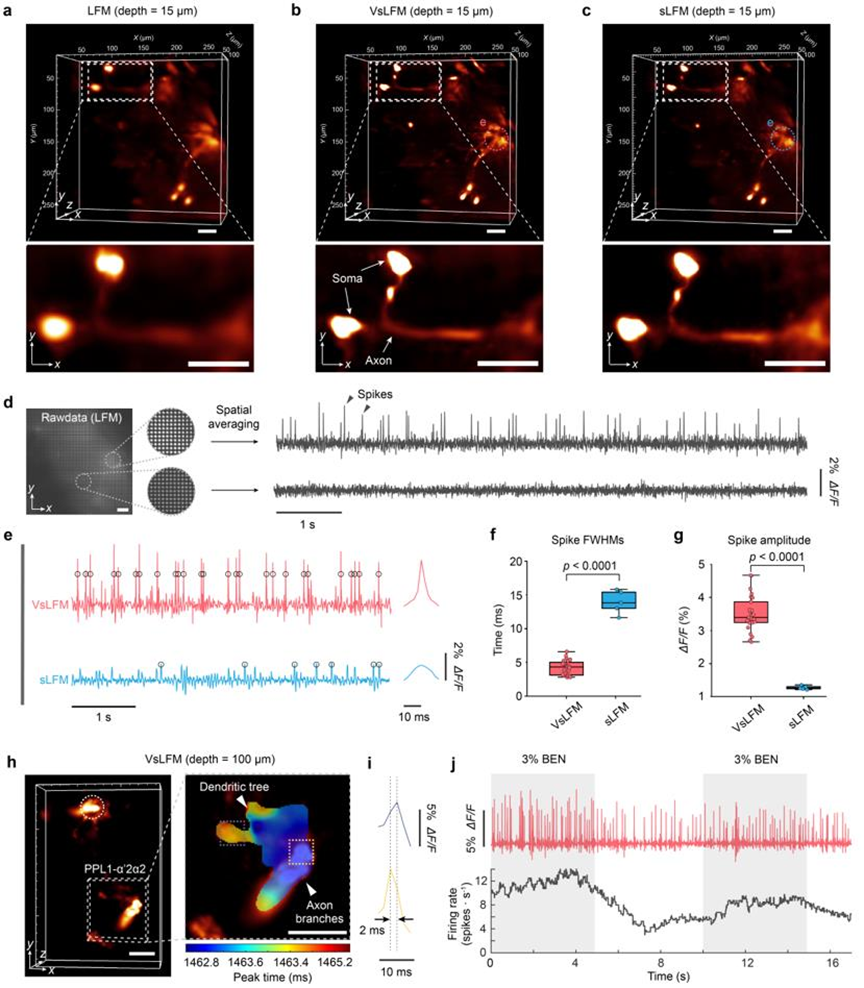
Figure 5. Recordings of ultra-high spatiotemporal resolution 3D voltage responses in multiple brain regions of Drosophila at 500VPS
It's worth mentioning that this paper also released the world's first light field microscopy super-resolution dataset, Bio-LFSR (https://doi.org/10.5281/zenodo.7233421), for free use by researchers in related fields, promoting the development of an open-source community for computational microscopy. It is believed that live computational imaging technologies, represented by VsLFM, will continue to improve and develop, driving the prosperity and progress of live research in life sciences and medicine. This will help humanity unveil the mysterious veil of the microscopic world within living organisms.
Dr. Lu Zhi from the Department of Automation at Tsinghua University and Liu Yu from the School of Electrical Automation and Information Engineering at Tianjin University are the co-first authors of this paper. Prof. Dai Qionghai from the Department of Automation, Institute for Brain and Cognitive Sciences, and Tsinghua-IDG/McGovern Institute for Brain Research at Tsinghua University, along with Assist. Prof. Wu Jiamin, and Professor Yang Jingyu from the School of Electrical Automation and Information Engineering at Tianjin University, are the co-corresponding authors. Several professors, postdoctoral researches and graduate students from Tsinghua University and Tianjin University made significant contributions to this work. This work was supported by the National Natural Science Foundation of China and the Ministry of Science and Technology.
Link: https://www.nature.com/articles/s41592-023-01839-6
References:
[1] Wu, J., Lu, Z., Jiang, D., et al. Iterative tomography with digital adaptive optics permits hour-long intravital observation of 3D subcellular dynamics at millisecond scale. Cell 184, 3318-3332 (2021).
[2] Lu, Z., Liu, Y., et al. Virtual-scanning light-field microscopy for robust snapshot high-resolution volumetric imaging. Nat. Methods (2023). https://doi.org/10.1038/s41592-023-01839-6.