As the field of artificial intelligence opens a new chapter with high-computing power and large models, the question of how to achieve efficient and precise training of large-scale neural networks has become a hot topic in international frontier research.
The research team led by Professor Lu Fang from the Department of Electronic Engineering and the team led by Academician Qionghai Dai from the Department of Automation at Tsinghua University have pioneered a new approach by creating an Fully forward mode intelligent Optical Training architecture. They have developed the "Taichi-II" optical training chip, breaking away from the traditional dependence on GPU offline modeling in optical computing systems, enabling efficient and precise training of large-scale neural networks.
This research result, titled "Fully forward mode training for optical neural networks," was published online in the journal Nature on the evening of August 7, Beijing time.
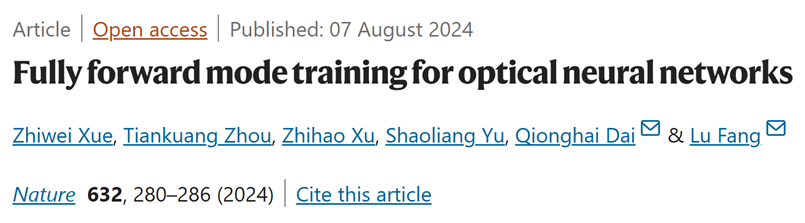
The Department of Electronic Engineering at Tsinghua University is the first unit for the paper, with Professor Lu Fang and Professor Qionghai Dai as corresponding authors. PhD student Zhiwei Xue and postdoctoral researcher Tiankuang Zhou from the Department of Electronic Engineering are co-first authors, with participation from PhD student Zhihao Xu from the Department of Electronic Engineering and Dr. Shaoliang Yu from the Zhijiang Laboratory. This project was supported by the Ministry of Science and Technology of China, the National Natural Science Foundation of China, the Beijing National Research Center for Information Science and Technology, and the Tsinghua University-Zhijiang Laboratory Joint Research Center.
Nature reviewers noted in their review that "the ideas presented in this paper are very innovative, and the training process of such optical neural networks (ONNs) is unprecedented. The proposed method is not only effective but also easy to implement, making it a promising tool for training optical neural networks and other optical computing systems."
Ingenious Use of Symmetry to
Help Optical Computing Break Free from GPU Dependence
In recent years, intelligent optical computing, characterized by high computing power and low energy consumption, has gradually stepped onto the stage of computational development. The advent of the general-purpose intelligent optical computing chip "Taichi" is a reflection of this trend, as it was the first to push optical computing from principle verification to large-scale experimental applications. With a system-level energy efficiency of 160 TOPS/W, it brought hope for "inference" in large-scale complex tasks, but it could not unleash the "training potential" of intelligent optical computing.
Training and inference are the two cornerstone capabilities of AI large models, and both are indispensable. Compared to model inference, model training requires even greater computing power.
However, existing optical neural network training is heavily dependent on GPUs for offline modeling and requires precise alignment of physical systems. As a result, the scale of optical training has been significantly limited, and the advantages of high-performance optical computing seem to be locked in invisible shackles.
At this juncture, the teams of Lu Fang and Qionghai Dai found the "key" in "photon propagation symmetry," equating both forward and backward propagation in neural network training to forward propagation of light.
According to the paper's first author, PhD student Zhiwei Xue from the Department of Electronic Engineering, under the Taichi-II architecture, backward propagation in gradient descent is transformed into forward propagation of the optical system. Training of optical neural networks can thus be achieved with two forward propagation of data-error. These two forward propagation naturally align, ensuring accurate calculation of physical gradients. This high training precision can support large-scale network training.
Since back-propagation is not required, the Taichi-II architecture no longer relies on electronic computing for offline modeling and training. As a result, the training of optical neural networks will no longer be tightly constrained by GPUs, and the precise and efficient optical training of large-scale neural networks is finally within reach.
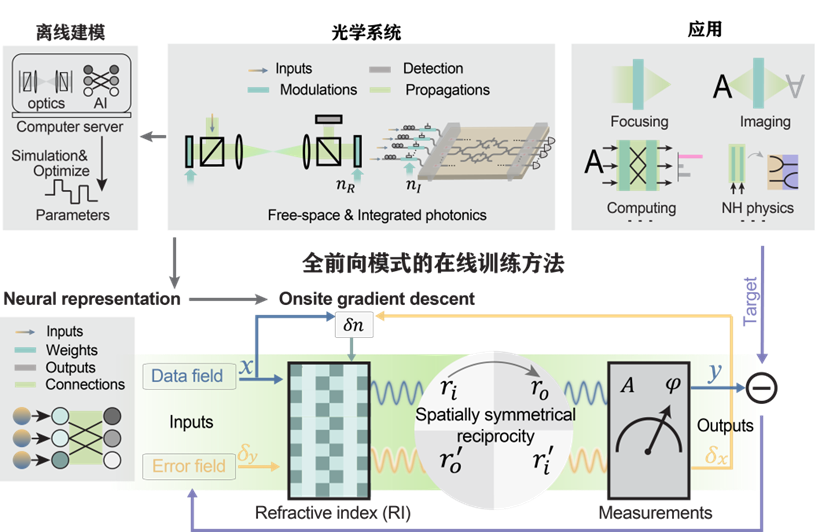
Fully forward mode Intelligent Optical Training Architecture
Efficient and Precise,
Intelligent Optical Training Can Achieve Anything
Using light as a medium for computation and constructing computing models through controllable propagation of light, optical computing inherently possesses the characteristics of high speed and low power consumption. Utilizing Fully forward mode propagation of light to achieve training can greatly enhance the speed and energy efficiency of optical network training.
The research shows that Taichi-II can train various optical systems and exhibits outstanding performance across different tasks.
Large-scale Learning: It has resolved the contradiction between computational precision and efficiency, increasing the training speed of optical networks with millions of parameters by an order of magnitude and improving the accuracy of representative intelligent classification tasks by 40%.
Intelligent Imaging in Complex Scenarios: It achieved intelligent imaging with a kilohertz frame rate, increasing efficiency by 2 orders of magnitude.
Topological Photonics: Without relying on any model priors, it can automatically search for non-Hermitian exceptional points, providing a new approach for the efficient and precise analysis of complex topological systems.
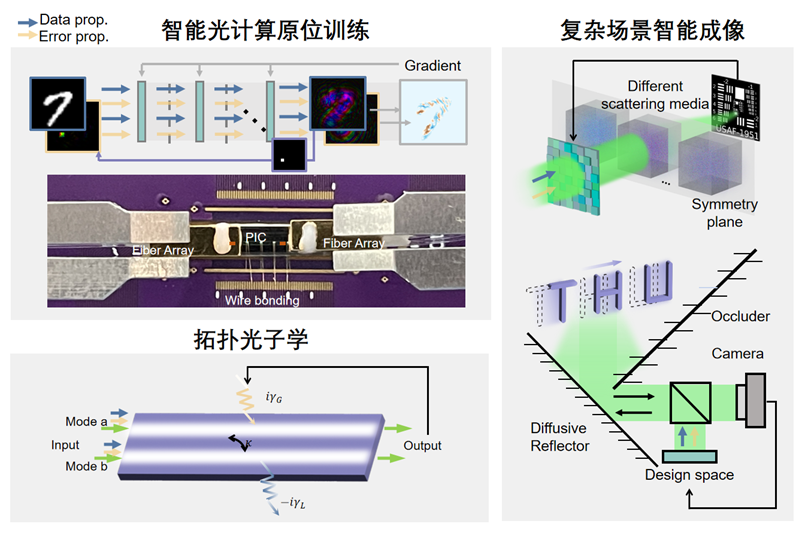
General Intelligent Optical Training Empowering Complex Systems
Join Forces with Taichi to
Propel AI Optical Computing Forward
The emergence of Taichi-II further reveals the immense potential of intelligent optical computing following the Taichi-I chip.
Like the duality of Yin and Yang, Taichi I and II respectively achieve high-energy-efficiency AI inference and training; Like the harmony of Yin and Yang, Taichi I and II together constitute the complete lifecycle of large-scale intelligent computing.
Lu Fang stated: "As we say, 'Determining the Way of Taichi, Harmonizing the Opposing Forces of the Universe,' we describe this dialectical and collaborative architecture of the Taichi series in this way. We believe they will jointly inject new momentum into the development of computing power for future AI large models, building a new foundation for optical computing."
Based on the prototype, the research team is actively moving towards the industrialization of intelligent optical chips, with application deployments in various edge intelligence systems.
It can be expected that through the relentless efforts in the field of optical computing, including the Taichi series, the intelligent optical computing platform is expected to open new paths for high-speed, high-energy-efficiency computing in AI large models, general artificial intelligence, and complex intelligent systems with lower resource consumption and marginal costs.