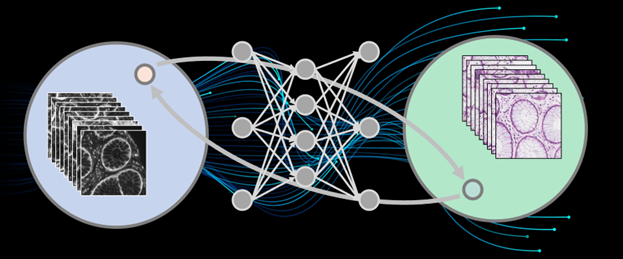
Optical microscopy is a crucial observation tool in life sciences and biomedicine. However, due to limitations of physical devices, imaging conditions, and sample preparation processes, direct microscopic images often fail to reveal sufficient sample information. In recent years, deep learning has made significant progress in computational imaging and image transformation. As a data-driven method, deep neural networks with numerous parameters can theoretically approximate any mapping from input to output domains. This offers a promising solution for image transformation in biomedical imaging. The goal of microscopic image transformation is to convert one type of image into another, revealing sample information and enabling feature extraction. This makes previously undetectable structures and potential biomedical features visible, aiding downstream biomedical analysis and disease diagnosis.
Currently, the superior performance of deep learning algorithms heavily relies on large amounts of paired training data. In the traditional supervised learning paradigm, obtaining the necessary paired ground truth requires extensive and labor-intensive registration and annotation. In microscopy, due to the high-speed dynamics of biological activities or incompatibility of imaging modes, as well as the irreversible nature of sample preparation, acquiring paired training data is often impractical in many scenarios. To advance the practical application of deep learning-based image transformation in biomedical imaging, it is crucial to overcome the dependency on paired training data.
The team led by Qionghai Dai from the Institute of Brain and Cognitive Sciences at Tsinghua University proposed an unsupervised content-preserving transformation for optical microscopy (UTOM). This method can learn the mapping relationship between two image domains without paired microscopy datasets, ensuring that the semantic information of images remains undistorted during transformation. UTOM achieves virtual pathological staining of human colorectal tissues, microscopic image restoration (denoising, isotropic resolution recovery, super-resolution reconstruction), and virtual fluorescence labeling, even in the absence of paired training data.
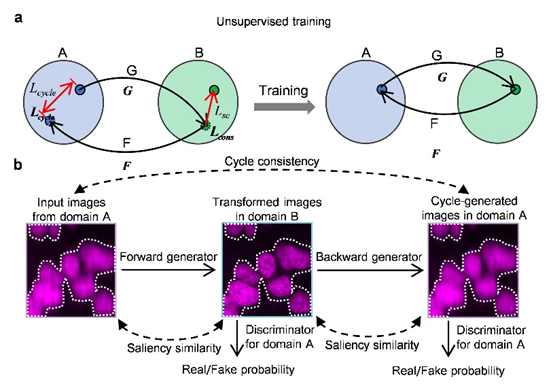
Figure 1. Principle of Unsupervised Microscopic Image Transformation Technology
Research Background
Current image transformations in microscopy are typically achieved in a supervised manner. Various network structures have been used for image transformations in recent years. U-Net, one of the most popular convolutional neural networks, has been applied to cell segmentation and detection, as well as microscopic image restoration. Specially designed convolutional neural networks can enhance optical imaging resolution and achieve imaging modality transfer. Additionally, Generative Adversarial Networks (GANs), a new framework based on adversarial optimization, simultaneously train generative and adversarial models, making transformed images more realistic. However, in application, these supervised learning methods face time-consuming and labor-intensive data collection and labeling errors. In microscopy, when live samples undergo rapid dynamic changes, the same scene cannot be captured twice by the imaging system; when sample preparation and imaging processes damage samples, they cannot be reused. These common scenarios prevent the acquisition of paired data, rendering traditional supervised learning methods inapplicable.
Cycle-Consistent Adversarial Networks (CycleGAN) were developed to achieve image style transfer in an unsupervised manner. CycleGAN can transform images from one domain to another without paired data, performing comparably to supervised methods. This framework has been used in style transfer of natural images and medical image analysis. In optical microscopy, some forward-looking studies have used CycleGAN to remove coherent noise in optical diffraction tomography and segment brightfield and X-ray computed tomography images.
Innovative Research
The proposed UTOM method enables unsupervised image content transformation when paired ground truth is missing. Based on CycleGAN, it introduces saliency constraints to address inherent content confusion, which is unacceptable in microscopic image transformations as it can mislead biomedical analysis and cause serious diagnostic errors. During cross-domain transformations, UTOM can locate and preserve image content, effectively avoiding semantic information distortion, thus significantly enhancing transformation accuracy. With this method, important semantic content in input images, such as gland lumens, goblet cells, intercellular spaces, and lymphocytes, can be clearly resolved.
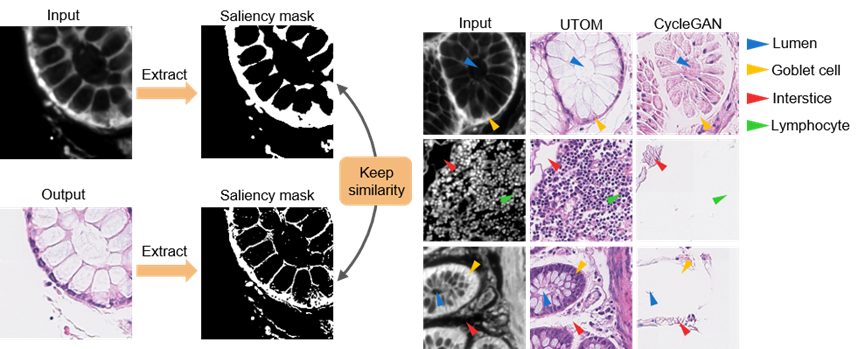
Figure 2. UTOM Effectively Avoids Image Content Confusion
This method has been applied to label-free pathological imaging, converting label-free autofluorescence images into standard Hematoxylin and Eosin (H&E) stained images. In diagnostics, H&E stained histopathological slices provide rich phenotypic information about samples and are the current "gold standard" for tumor diagnosis. However, traditional histopathological examination requires staining, involving many complex chemical steps and toxic reagents, hindering intraoperative diagnosis and rapid cancer screening. Obtaining standard H&E stained images from label-free imaging methods has been a long-term goal for biomedical researchers. This paper presents a simple and feasible image transformation method: human tissue specimens are fixed and sectioned, with one group undergoing autofluorescence imaging without staining and the other undergoing standard H&E staining and brightfield imaging. The images acquired from these two imaging modes are unpaired due to different structures. Nevertheless, using UTOM, high-fidelity virtual fluorescence staining can be achieved even without paired ground truth, resulting in medically significant standard H&E stained images.
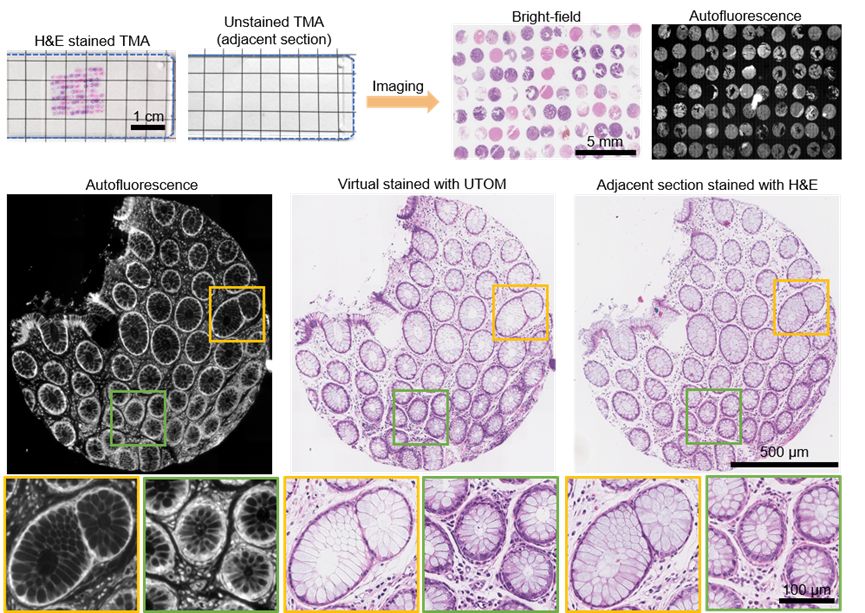
Figure 3. Unsupervised Learning Achieves Virtual Staining of Pathological Slices with Non-Paired Slices
Additionally, due to the fragility of live samples and the presence of photobleaching and phototoxicity, fluorescence images usually have low signal-to-noise ratios. The dynamic nature of samples makes obtaining paired ground truth extremely difficult. Using this method, effective denoising networks can still be trained without paired ground truth. Similarly, this method has been demonstrated for axial resolution recovery, wide-field super-resolution reconstruction, and virtual fluorescence labeling.
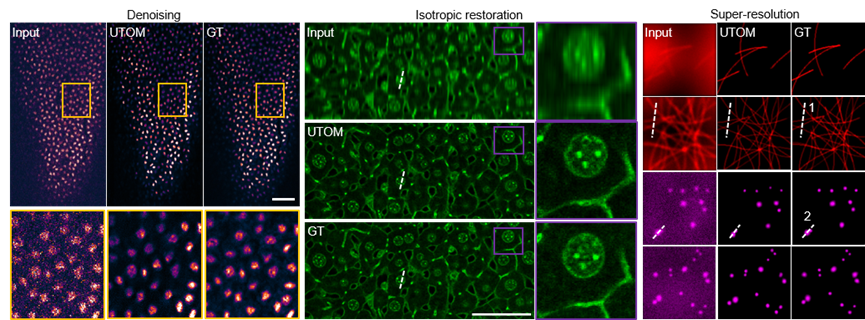
Figure 4. Unsupervised Learning Achieves Fluorescence Image Restoration
Applications and Prospects
The proposed method is expected to promote a paradigm shift in deep neural network training in the field of microscopy from traditional supervised learning to unsupervised methods. In optical microscopy, unsupervised learning has unique advantages, completing tasks that supervised learning cannot handle, especially when samples have rapid dynamic characteristics or imaging processes are destructive. Additionally, combining the physical model of the imaging process will further reduce network parameters and enhance performance. Once the dependency on paired training data is eliminated, more challenging microscopic image transformation tasks will become possible.
Paper link: https://www.nature.com/articles/s41377-021-00484-y