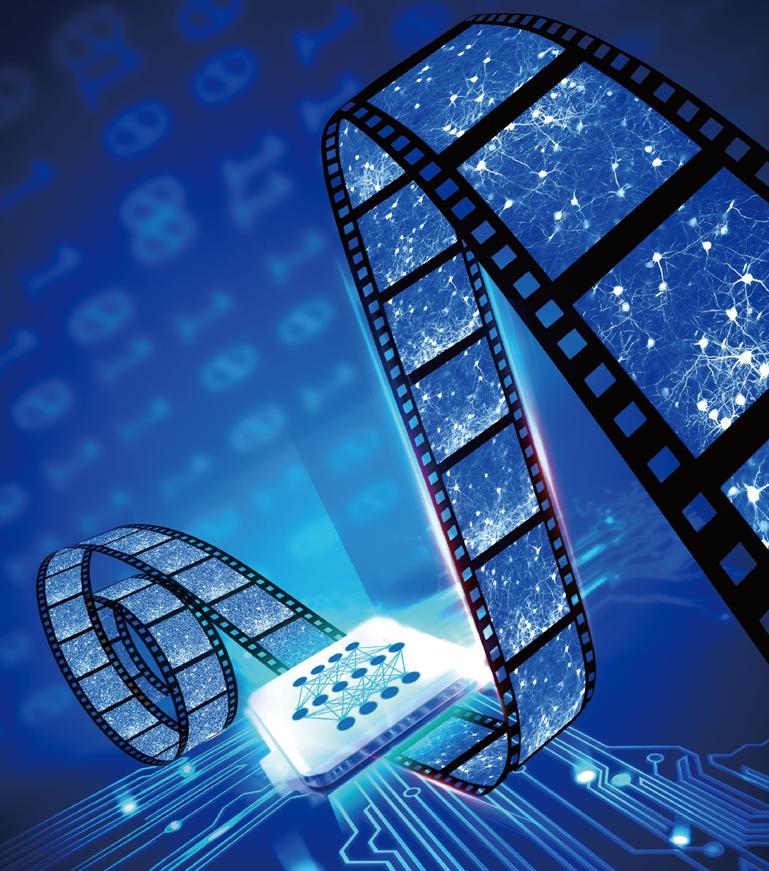
Calcium imaging allows parallel recording of neural activity in living organisms at single-cell resolution, offering great potential for unraveling the mechanisms of information propagation, integration, and computation in neural circuits. Achieving high signal-to-noise ratio (SNR) calcium imaging data is crucial for accurate neural function analysis. However, the low peak accumulation and rapid dynamics of in vivo calcium transients result in insufficient photon capture by detectors, hindered by the quantum nature of light and Poisson noise limits. Low SNR in calcium imaging has long troubled neuroscientists, forcing compromises in imaging speed, resolution, or tissue viability to improve SNR, significantly limiting advancements in life sciences.
Breakthrough in Calcium Imaging Denoising
On August 16, 2021, Nature Methods published a study by Qionghai Dai's team from the Institute of Brain and Cognitive Sciences of Tsinghua University. The title is "Reinforcing neuron extraction and spike inference in calcium imaging using deep self-supervised denoising."
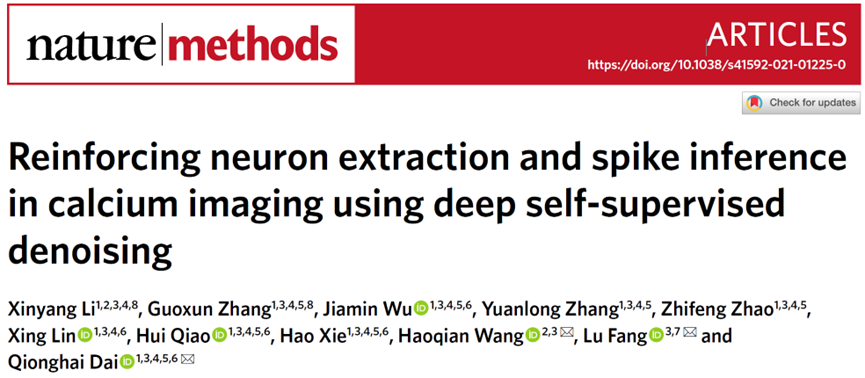
This study proposes a self-supervised learning method (DeepCAD) for denoising calcium imaging, training the network using only a single low-SNR calcium imaging video sequence. The high-speed, non-repetitive nature of neuronal calcium activity prevents the acquisition of ground truth required for traditional supervised learning. This self-supervised strategy eliminates the dependency on ground truth, achieving a breakthrough in calcium imaging denoising. DeepCAD employs a 3D network structure to fully utilize the spatiotemporal correlation of neural activity, enhancing the calcium imaging SNR by over tenfold, overcoming photon noise limits. This method was used to observe calcium dynamics in the neural fiber network and neuronal populations in the mouse cortex, achieving high SNR calcium imaging under low excitation power, and is widely applicable to various calcium imaging systems.
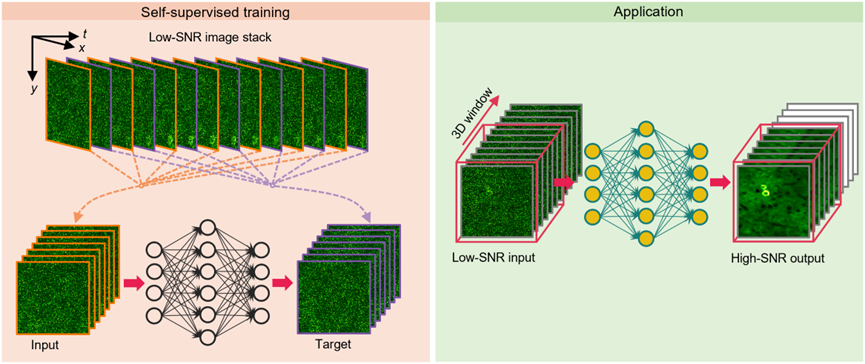
Figure 1. Principle and application of DeepCAD
Validation and Applications of DeepCAD
To validate the accuracy and reliability of this method, the authors built a specialized two-photon microscope, where the emitted fluorescence photons from the sample were split into two parts in a 1:10 ratio at the detection end, and the signals were synchronously acquired. The low-SNR image served as the raw data input to the network, while the high-SNR image was used to validate the output. DeepCAD was first applied to denoise calcium imaging of the neural fiber network in Layer 1 of the mouse cortex. The results showed that the SNR of the denoised data improved by over tenfold, and the calcium activity initially buried in noise was accurately recovered.
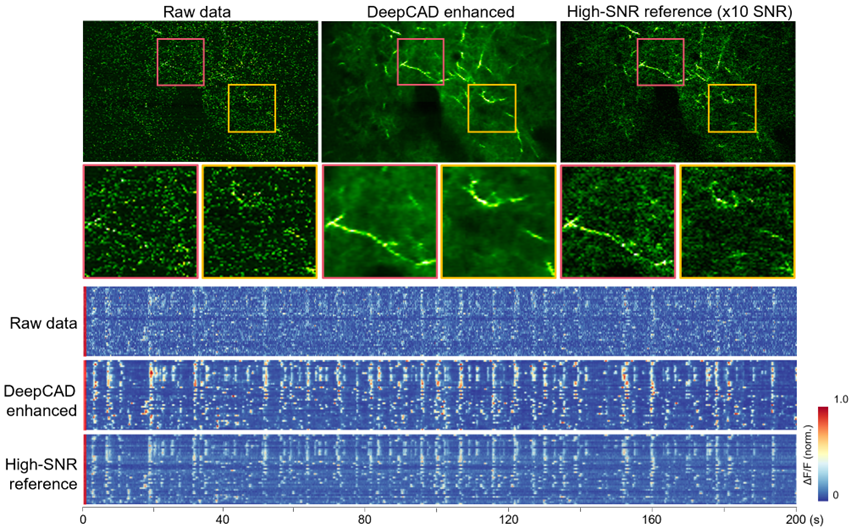
Figure 2. DeepCAD recovers neural fiber network calcium dynamics buried in noise, enhancing SNR by over tenfold
Denoising large neural populations with good performance
DeepCAD was also applied to observe calcium activity in neuronal populations in Layer 2/3 of the mouse cortex. The denoised calcium imaging data exhibited very high SNR, allowing clear identification of neuronal morphology and distribution from single frames. By effectively removing detection noise, the accuracy of somatic calcium signals was significantly improved. Quantitative analysis showed that DeepCAD-enhanced calcium imaging data markedly improved the accuracy of neuron extraction and spike inference, helping to fully reveal neural circuit activity and avoid information loss due to noise.
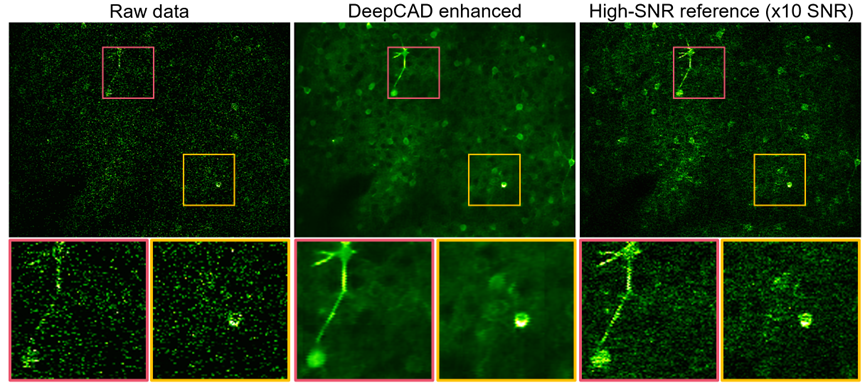
Figure 3. DeepCAD denoises calcium imaging of neuronal populations, accurately parsing neuronal activity
Code link: https://github.com/cabooster/DeepCAD
Paper Link: https://www.nature.com/articles/s41592-021-01225-0