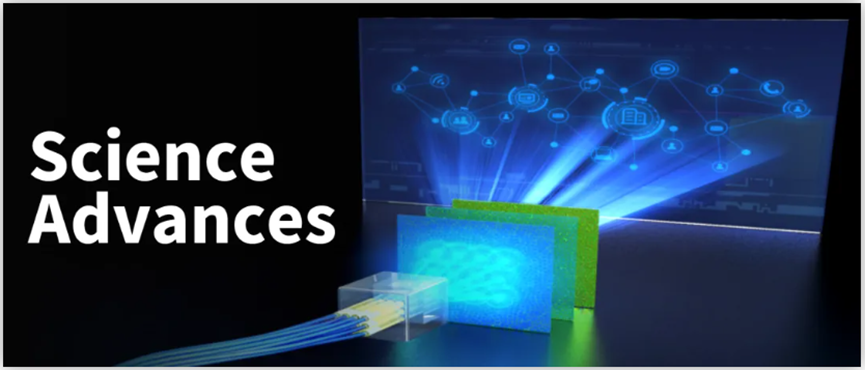
With the development of an intelligent society, image transmission has become a fundamental need in various fields of daily life. For instance, streaming a Blu-ray movie requires transmission throughput of several gigabits per second. Transmission links need to support such high throughput for numerous terminals simultaneously, posing significant challenges for image transmission. Additionally, image transmission often demands high visual quality, necessitating pre-processing and post-processing tasks such as compression and error correction, which involve substantial computational operations.
However, despite over 95% of the world's digital information being transmitted through optical fibers, image processing still primarily relies on electronic processors. Processing large volumes of optical image signals, such as encryption and compression, places a heavy burden on current electronic computing platforms. The frequency gap between the most advanced electronic circuits and optical fiber transmission lines spans several orders of magnitude, making it one of the most time-consuming aspects of high-throughput transmission.
The recent rise of intelligent photonic computing is seen as a potential solution to overcome electronic bottlenecks by processing images directly in the photonic domain. Yet, existing all-optical neural networks mainly focus on classification tasks such as recognizing handwritten digits and letters. Image transmission processing requires not only feature extraction but also reconstruction after compression and encryption.
Recent advancements in electronic generative models have demonstrated their capability in realistic image reconstruction. For example, Variational Autoencoder (VAE) models can perform controlled transformations between large-scale datasets and low-dimensional representations (latent space) and have been applied in data encoding and generation. However, current photonic intelligent processors cannot achieve all-optical end-to-end generative neural networks, thus failing to completely eliminate the bottleneck of optoelectronic conversion and realize ultra-low latency complex image processing during transmission.
Recently, Academician Dai Qionghai and Associate Professor Fang Lu’s team at Tsinghua University proposed an unsupervised photonic variational autoencoder, called the Photonic Encoder-Decoder (PED). This innovation achieves all-optical end-to-end processing in image transmission and demonstrates the first all-optical generative neural network.
Compared to advanced Central Processing Units (CPUs), the Photonic Encoder-Decoder reduces system latency in image transmission by more than four orders of magnitude. On medical datasets, the throughput of the Photonic Encoder-Decoder is two orders of magnitude higher than the common PAM-8 method and 87 times higher than traditional DCT processing methods.
The results were published in Science Advances under the title “Photonic unsupervised learning variational autoencoder for high-throughput and low-latency image transmission.” Ph.D. students Chen Yitong and Zhou Tiankuang from Tsinghua University are the co-first authors of the paper.
It is worth noting that in September 2022, the news program Focus Report covered related research achievements and other significant scientific progress from this research group.
Principle of the Photonic Encoder-Decoder
The principle architecture of the proposed Photonic Encoder-Decoder is shown in Figure 1. Input information is encoded into a latent space with an optical artificial neural network encoder and then coupled into a single-mode fiber bundle accordingly. The noise during transmission is modeled as variations in the latent space based on the VAE architecture. The optical artificial neural network decoder reads and decodes the transmitted latent space, i.e., the Gaussian speckle pattern after the collimating lens, faithfully reconstructing the input information. The latent space highly encrypts the input information, preventing eavesdropping and ensuring transmission security.
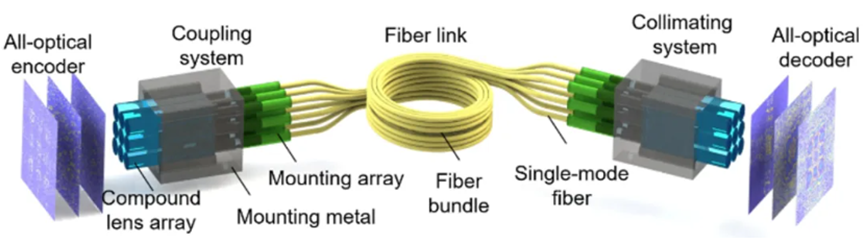
In the all-optical encoder composed of trainable diffraction layers, the Photonic Encoder-Decoder passively couples the optical field into the fiber bundle through a lens array and uses an all-optical decoder to decode the latent space back to the original data. Each lens in the lens array couples a corresponding region of the optical field into a single-mode fiber in the bundle. The coupling efficiency depends on the characteristics of the optical field, fiber, and lens. The optical field in the fiber can be considered a linear superposition of different spatial frequencies with respective coupling coefficients.
General and Specialized Transmission Modes
The Photonic Encoder-Decoder supports two working modes: general transmission mode and specialized transmission mode. The general transmission mode provides basic secure and noise-resistant transmission for arbitrary images, as shown in Figure 2. Experiments and simulations show that the general transmission mode achieves similar or lower error rates compared to existing error-correcting codes while optimizing computational power consumption and speed by several orders of magnitude.
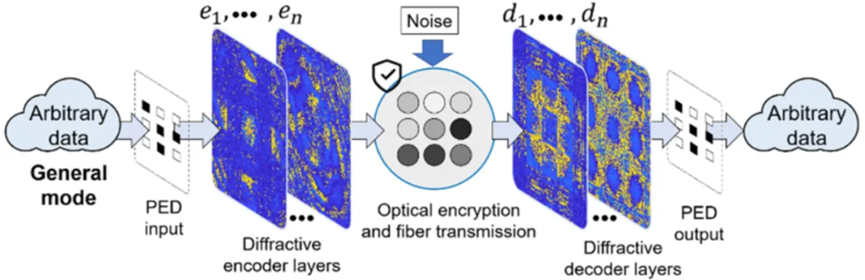
When some prior information is available, the specialized transmission mode allows significant compression to improve transmission throughput. This prior information is not limited to labels or other information; the Photonic Encoder-Decoder can be trained unsupervised to find an optimal encoding compression latent space.
Encryption and Throughput Enhancement
Figure 3 shows the experimental results of encryption on binary images (Tsinghua University logo) using the Photonic Encoder-Decoder. The information transmitted through the fiber bundle, which might be intercepted, is encrypted into unrecognizable images with a significantly higher error rate than the reconstructed results (annotated in the top left corner of each small image). Letters (“VER”), numbers (“191”), and Chinese characters are effectively encrypted and unrecognizable.
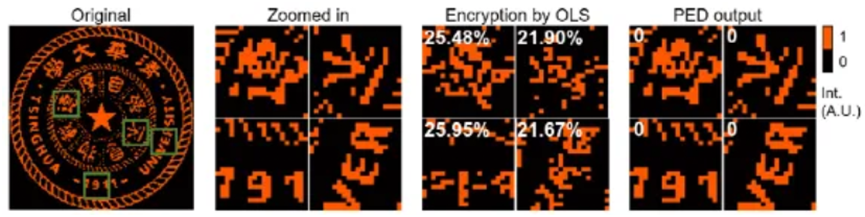
The authors further validated the performance of the Photonic Encoder-Decoder with clinically collected CT images. As shown in Figure 4, the Photonic Encoder-Decoder successfully reconstructed the shapes and features of adrenal glands (second and fourth columns) at compression ratios of 31 and 87 times, while DCT failed to retain any details at the same compression ratios (third and fifth columns). Even at a high compression ratio (87 times), the reconstruction quality of the Photonic Encoder-Decoder remained consistently high, easily distinguishing different categories (class 1 and class 2), which DCT could not achieve (fifth column). This not only demonstrates the Photonic Encoder-Decoder's powerful transmission and compression capabilities but also its potential for intelligent computing such as disease diagnosis and semantic understanding during transmission.
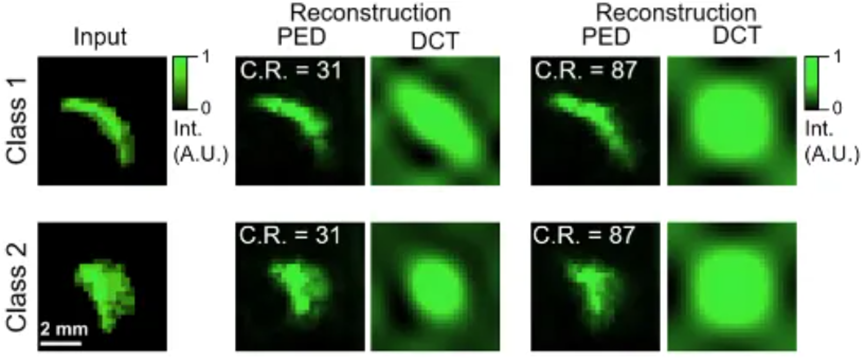
Summary and Outlook
The authors also calculated the end-to-end system latency of the Photonic Encoder-Decoder in an optical communication system, including computation, optoelectronic conversion, and analog-to-digital converters. Compared to advanced CPUs (Intel Core i7 6500U CPU @2.5 GHz), the Photonic Encoder-Decoder reduces system latency by four orders of magnitude. It shows that the Photonic Encoder-Decoder optimizes the time-consuming bottleneck limiting throughput improvement, rather than parts that are already fast enough. Even compared to state-of-the-art GPUs with ultra-high computing speed (approximately 47 TOPS/S), the Photonic Encoder-Decoder still achieves an order of magnitude improvement in system latency.
By synergistically designing the encoder, decoder, and fiber system in the optical domain, the authors’ work establishes an intrinsic connection between unsupervised learning architectures and physical models of fiber communication systems, inspiring the next generation of all-optical communication systems with higher throughput and accuracy.
The authors believe that the proposed generative photonic computing system and end-to-end unsupervised photonic learning methods will promote a wide range of AI applications, including 6G, medical diagnostics, robotics, and edge computing.
Paper Information
Chen et al., Sci. Adv. 9, eadf8437 (2023)
https://doi.org/10.1126/sciadv.adf8437