In the three-dimensional space of cells and tissues, complex biological processes maintain a precise and orderly operation at all times, sustaining the basic functions of life activities. To observe dynamic biological processes from a three-dimensional perspective, the Nobel Prize in Chemistry winner Eric Betzig invented the lattice light-sheet microscope (LLSM), which significantly reduces the photobleaching and phototoxicity of live samples compared to traditional wide-field or confocal microscopes. The lattice light-sheet structured illumination microscope (LLS-SIM) combines LLSM with structured illumination (SIM), achieving an ideal balance between resolution, imaging speed, and imaging duration. However, the traditional LLS-SIM uses single-directional illumination, resulting in anisotropic spatial resolution, and prone to distortion in the non-super-resolution direction, limiting the precise detection of three-dimensional subcellular dynamics.
On April 29, 2025, the team from the Department of Automation at Tsinghua University, led by Dai Qionghai, and the research group of Li Dong from the School of Life Sciences, published a research paper titled "Fast-adaptive super-resolution lattice light-sheet microscopy for rapid, long-term, near-isotropic subcellular imaging" in the journal Nature Methods. This study proposes a meta-learning-driven reflective lattice light-sheet virtual structured illumination microscope (Meta-rLLS-VSIM) and the corresponding isotropic super-resolution reconstruction framework. Through technological innovations such as virtual structured illumination, mirror-enhanced dual-view detection, and Bayesian dual-view fusion reconstruction, without sacrificing the core imaging indicators such as imaging speed and photon cost, the one-dimensional super-resolution capability of LLS-SIM is extended to the XYZ three dimensions, achieving a near-isotropic imaging resolution of 120 nm in the lateral direction and 160 nm in the axial direction. Particularly, this work deeply integrates the meta-learning strategy with the system data acquisition process, enabling the completion of the adaptive deployment process from training data collection to the deep learning model in just 3 minutes, making the application of AI tools in actual biological experiments nearly "zero-threshold". The collaborative team conducted experiments on various specimens such as plant pollen tubes, mouse embryos, Caenorhabditis elegans embryos, and other eukaryotes in mitosis or interphase, verifying the imaging capabilities of Meta-LLS-VSIM in rapid, long-term, and multi-color three-dimensional super-resolution imaging tasks.
The virtual structured illumination achieves lateral isotropic super-resolution reconstruction.
LLS-SIM generates a structured light sheet illumination pattern in a single direction through the interference of two beams of light (Figure 1a), thereby improving the spatial resolution in a single dimension. However, limited by the orientation of the excitation objective lens optical axis, the generated structured light pattern cannot rotate like the standard SIM, resulting in anisotropic spatial resolution. To address this issue, the collaborative team proposes a virtual structured illumination scheme. Firstly, the LLS-SIM structured illumination mode is used to collect high and low-resolution data pairs to train a deep neural network (DNN) model for one-dimensional super-resolution inference (Figure 1b). Then, the trained DNN is applied to generate anisotropic one-dimensional super-resolution images in other directions. Finally, similar to the standard SIM reconstruction, multiple one-dimensional super-resolution images with different orientations are deconvolved and reconstructed through generalized Wiener filtering to obtain a lateral isotropic super-resolution volume stack (Figure 1c). Figures 1d-l show the comparison of the imaging effects of different methods on actin microfilaments, verifying the significant resolution improvement effect of the proposed virtual structured illumination imaging in the spatial and frequency domains.
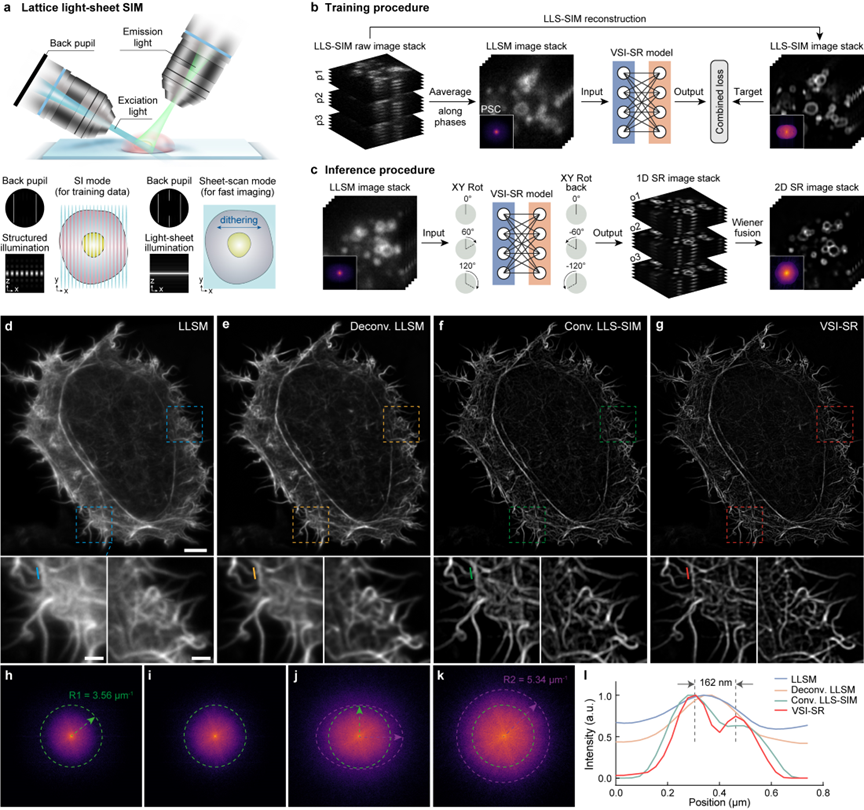
Figure 1 The virtual structured illumination achieves lateral isotropic super-resolution reconstruction
The meta-learning-driven model enables rapid adaptive deployment
Due to the morphological diversity of subcellular biological structures and the limited representational capacity of DNN, the current deep learning super-resolution methods usually require training a dedicated model for each specific biological structure to achieve the best inference effect, and each model training requires hundreds to thousands of pairs of high-quality data, taking several hours to several days, making it difficult to be widely used in daily imaging experiments of various biological samples. To this end, the collaborative team proposes a meta-learning solution, that is, regarding the super-resolution reconstruction problems of different biological samples and signal-to-noise ratios as separate sub-tasks. Firstly, a general meta-model is trained through existing data. Different from traditional supervised learning or pre-trained models, the general meta-model aims to learn the common needs of different sub-tasks and find convergence points that can be quickly transferred to different tasks in the parameter space. Finally, in practical applications, only a small amount of data set and gradient update are required to quickly transfer to the model parameters adapted to the new task (Figures 2a-d).
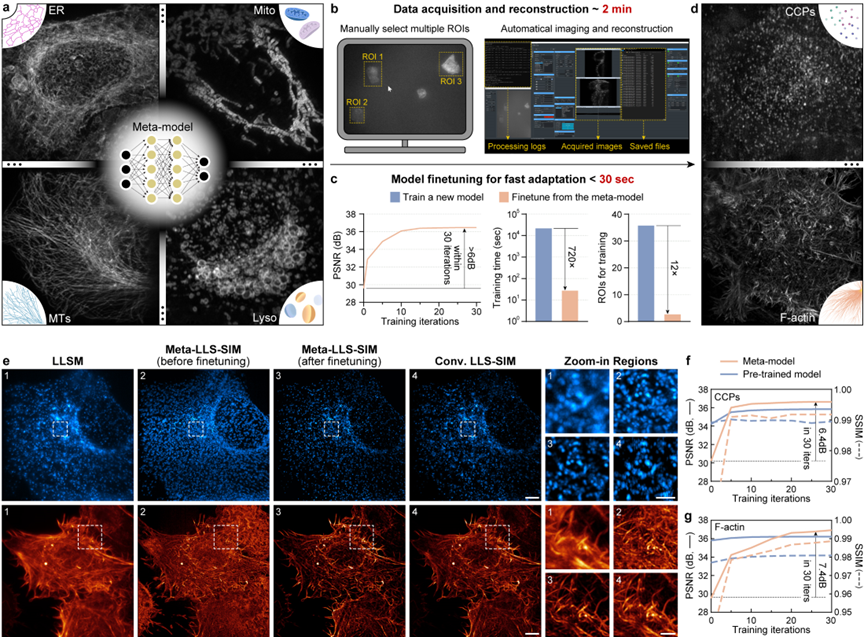
Figure 2 The meta-learning-driven model enables rapid adaptive deployment
To fully exert the performance of the meta-model, the research team endows the constructed LLS-SIM system with the rapid adaptive deployment capability driven by meta-learning by simplifying the automatic data acquisition, data preprocessing, and meta-fine-tuning processes. In practical applications, the user only needs to select 3 cell regions in the control software window, and the subsequent data acquisition and all computing processes (including LLS-SIM reconstruction, data enhancement, and meta-fine-tuning) will be automatically executed by the system. The entire data acquisition process takes approximately 2 minutes, while the model fine-tuning process (the peak signal-to-noise ratio of the reconstructed image is increased by more than 6 dB) using a single graphics card takes less than 30 seconds, and the required data volume is 12 times less than that of standard supervised learning, and the speed is 720 times faster (as shown in Figure 2c).
Figure 3 Video of meta-learning-driven fast adaptation process
The collaborative team used two new structures, clathrin-coated pits (CCP) and actin microfilaments (F-actin), to evaluate the fine-tuning effect of the meta-model (as shown in Figures 2e and f). It was found that the initial meta-model only requires one fine-tuning iteration to remove most of the artifacts, and the loss function can converge within 30 iterations, significantly improving the super-resolution reconstruction ability for the two structures. Combined with virtual structured illumination, the proposed method can eliminate the reconstruction artifacts of anisotropic LLS-SIM images and clearly distinguish the CCP distribution location and the densely intertwined structural details of F-actin.
Meta-rLLS-VSIM achieves near-isotropic super-resolution imaging
Virtual structured illumination can effectively extend the one-dimensional super-resolution capability to the two-dimensional plane, but it cannot improve the axial resolution. In recent years, several deep learning-based methods have directly improved the axial resolution through data-driven methods in the absence of ground truth using self-learning or Cycle-GAN. However, the axial resolution is usually more than three times worse than the lateral resolution, resulting in severe underdetermination of these methods, making it difficult to ensure the correctness of the reconstruction.
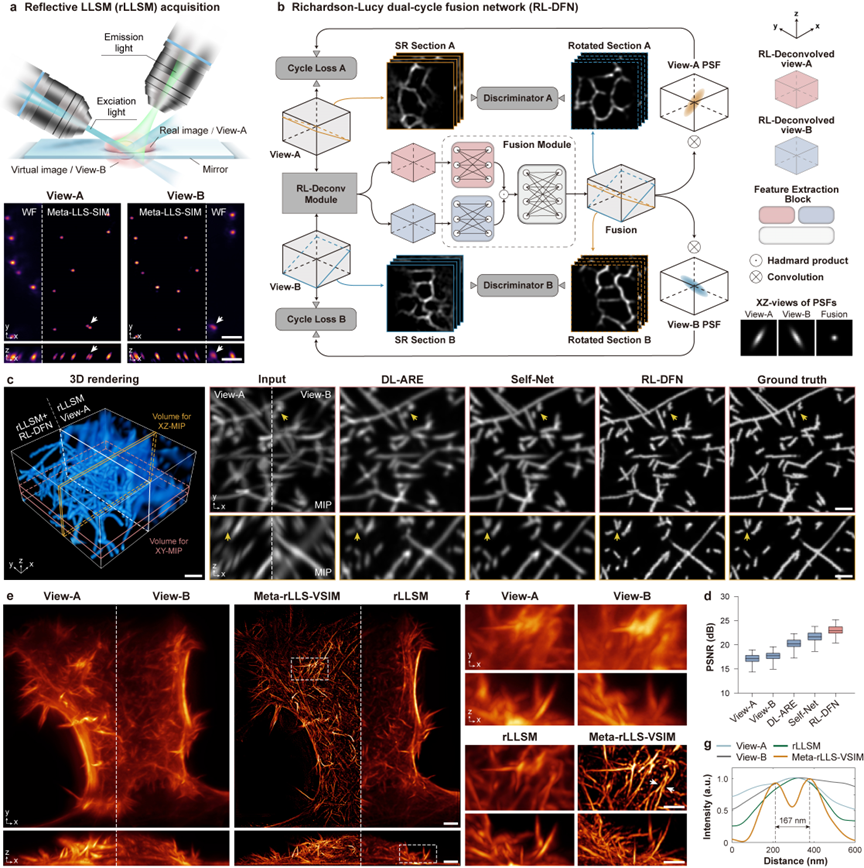
Figure 4 Meta-rLLS-VSIM achieves near-isotropic super-resolution imaging
To more reasonably improve the axial resolution of Meta-LLS-VSIM, the collaborative team adopts a mirror-enhanced dual-view detection scheme to simultaneously obtain two perspective information with complementary resolutions (Figure 3a), and designs a fusion algorithm - Richardson-Lucy double-cycle fusion network (RL-DFN), integrating multi-perspective RL iterations and the system point spread function (PSF) prior into the neural network architecture and loss function design (Figure 3b). The effective fusion of dual-view information makes the axial resolution improvement more reasonable from the physical essence. Compared with other data-driven algorithms, RL-DFN can restore the sample details more accurately with isotropic resolution (Figures 3c and d).
Integrating the above technological innovations, the collaborative team has constructed a complete three-dimensional isotropic reconstruction framework, including fluorescence background suppression, image volume stack de-tilting, lateral isotropic reconstruction, dual-view separation and registration, and RL-DFN dual-view fusion, ultimately achieving a near-isotropic imaging resolution of 120 nm in the lateral direction and 160 nm in the axial direction (Figures 3e-g), and the volume resolution is 15.4 times higher than that of the traditional LLSM (2.3 times in the lateral direction and 2.9 times in the axial direction).
Meta-rLLS-VSIM achieves rapid five-dimensional super-resolution live cell imaging
To demonstrate the rapid five-dimensional (wavelength channel - XYZ three-dimensional space - time) super-resolution live cell imaging capability of Meta-rLLS-VSIM, the collaborative team conducted long-term super-resolution observations on large-volume and thick samples such as mouse embryos (Figure 4a), plant pollen tubes (Figures 4b and c), and C. elegans embryos (Figures 4d-g). With the physical optical tomography of lattice light-sheet illumination and the near-isotropic three-dimensional super-resolution ability, Meta-rLLS-VSIM clearly reveals biological processes such as the polar growth at the tip of the pollen tube and the plasma membrane fusion during the embryonic development of C. elegans.
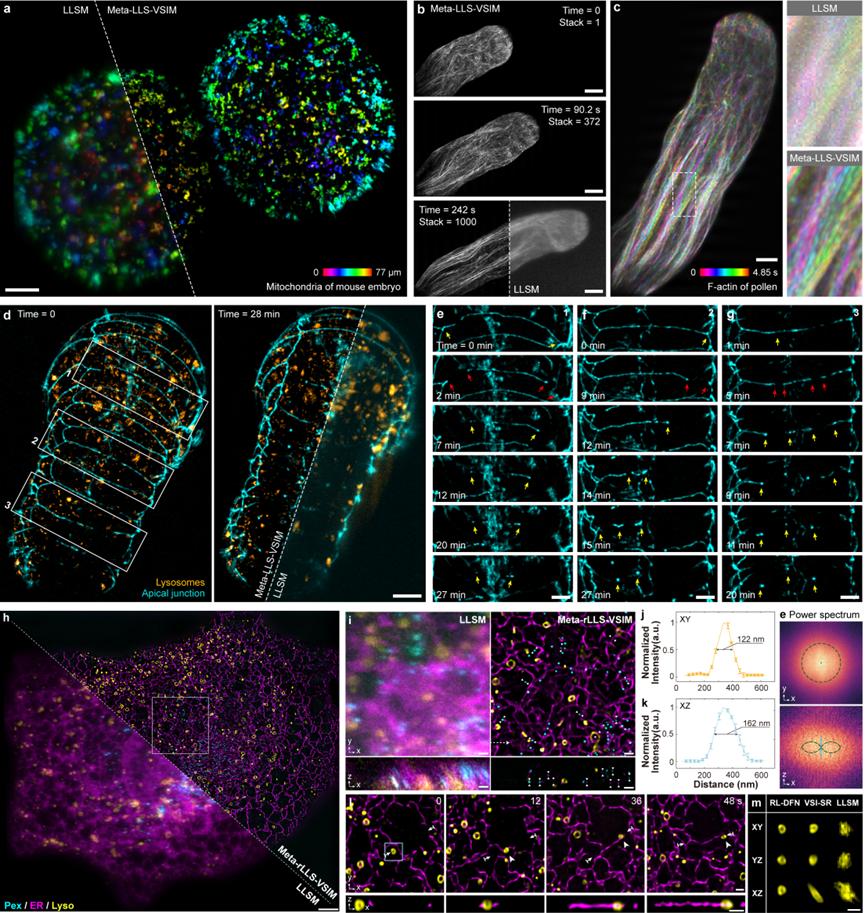
Figure 5 Meta-rLLS-VSIM achieves rapid five-dimensional super-resolution live cell imaging
Furthermore, the collaborative team used Meta-rLLS-VSIM to perform rapid (a set of three-channel complete cell data is captured every 8 seconds), long-term (>800 time points), and near-isotropic super-resolution imaging on intact COS-7 cells (Figures 4h-m), and conducted an accurate quantitative study on the distribution patterns of different organelles in the three-dimensional space and their spatiotemporal cooperative interaction mechanism with the cytoskeleton. Benefiting from the high spatiotemporal resolution and long-period observation window, the collaborative team observed new phenomena such as "hitchhiking" between microtubules and lysosomes, and the division of mitochondria under the mechanical force generated by the movement of lysosomes, verifying the potential of Meta-rLLS-VSIM in discovering new biological phenomena and mechanisms.
Figure 6 Imaging performance comparison between Meta-rLLS-VSIM and conventional LLSM
In summary, Meta-rLLS-VSIM showcases a significant improvement in imaging performance through the hardware upgrade of the imaging system with the mirror-enhanced dual-view lattice light-sheet microscope and the meta-learning rapid adaptive deployment mode, as well as the artificial intelligence algorithm innovation of virtual structured illumination and the RL double-cycle fusion network. The emergence of Meta-rLLS-VSIM provides a new technical path for the development of basic disciplines such as cell biology and neuroscience, and is expected to assist life science researchers in discovering, understanding, and exploring the rich and colorful biological phenomena from a more comprehensive multi-dimensional perspective.
Qiao Chang, a postdoctoral fellow at the Department of Automation, Tsinghua University, Ziwei Li, an associate researcher at Fudan University, Zongfa Wang, a postdoctoral fellow at the Institute of Biophysics, Chinese Academy of Sciences, Yuhuan Lin, a doctoral student, and Chong Liu, a postdoctoral fellow at École Polytechnique Fédérale de Lausanne, are the co-first authors of this paper. Professor Li Dong from the School of Life Sciences, Tsinghua University, and Academician Dai Qionghai from the Department of Automation, Tsinghua University, are the co-corresponding authors of this paper.