Professor Qionghai Dai’s research group from Department of Automation, Tsinghua University, devised a universal microscopic image denoising and super-resolution processing framework, named ZS-DeconvNet. The related study was published in Nature Communications on May, 16th, 2024, entitled “Zero-shot learning enables instant denoising and super-resolution in optical fluorescence microscopy”.
Microscopic image processing technology based on deep learning, including image super-resolution and denoising, can improve image quality without increasing the cost of in vivo imaging experiments, and is a powerful tool for promoting life science research. Existing methods rely on a large number of low-resolution/SNR to high-resolution/SNR microscopic image data pairs for learning, but due to the high dynamics of living biological samples and the diversity of biological structure types, it is impractical to collect a large amount of high-quality training data for each biological structure. These factors seriously hinder the use of existing methods in daily biological experiments.
Based on the noise model of microscopic images and the zero-shot learning theory, this study proposed a zero-shot universal microscopic image processing framework ZS-DeconvNet, and developed the corresponding one-click microscopic image processing software. ZS-DeconvNet can use as few as a single low-resolution/signal-to-noise ratio image and train in an unsupervised manner, stably improving the resolution of microscopic images to more than 1.5 times the diffraction limit, and the working fluorescence intensity is 10 times lower than the traditional super-resolution imaging conditions. The proposed ZS-DeconvNet is physical, universal, robust, and easy to use. Compared with the traditional deconvolution method based on iterative optimization, the data throughput is increased by more than 100 times. It is compatible with various imaging modalities including total internal reflection microscopy, three-dimensional wide-field microscopy, lattice light sheet microscopy, confocal microscopy, and multimodal structured illumination super-resolution microscopy, providing a powerful and reliable tool for the development of basic research in brain science, cell biology, etc.
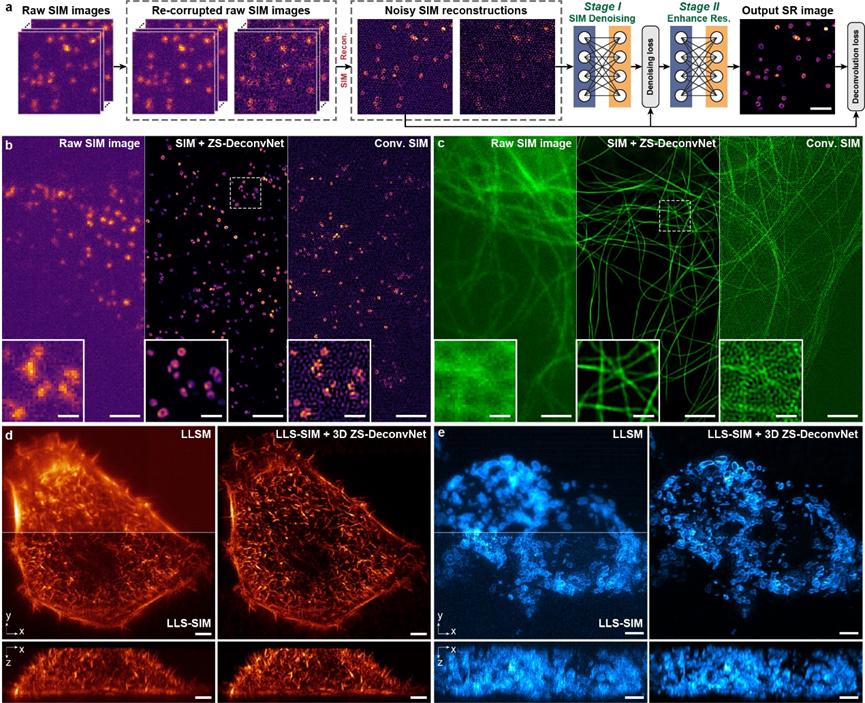
Figure | Schematic of ZS-DeconvNet framework and representative results