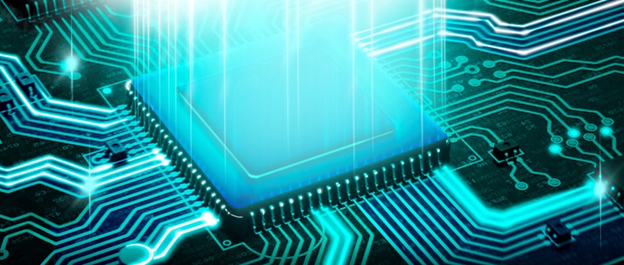
Artificial intelligence (AI) technology, represented by deep learning, has brought huge changes to our daily life in many fields, including autonomous driving, medical diagnosis, and voice translation. With the rapid development of deep learning algorithms and the continuous increase in the scale of artificial neural networks, there is an urgent need to continuously improve the computing speed and energy efficiency of computing processors. Using traditional electronic computing methods has become increasingly difficult to meet the future demands of AI for processor’s computing performance. In recent years, based on the disruptive advantages of optical computing with high speed, low power consumption, and high parallelism properties, the construction of optical neural networks and intelligent optoelectronic fused computing processors has become a research hotspot at the forefront of information technology.
However, the practical application of the existing optoelectronic intelligent computing technology still faces the following challenges: (1) Model complexity. Because of the low complexity of existing optical neural network models and the difficulty in implementing optical nonlinearity, the performance largely lags behind their electronic counterparts; (2) Error correction. Since the error of the optical computing system is positively correlated with the system complexity, the error correction algorithm is very important for building a large-scale intelligent optical computing system. However, the universal error correction method is still lacking so far; (3) Reconfigurability. Most of the existing optical neural network structures are difficult to be reconfigured with limited applications. Furthermore, large-scale network parameter programming is time-consuming and difficult to be calibrated due to the use of complicated optical effects.
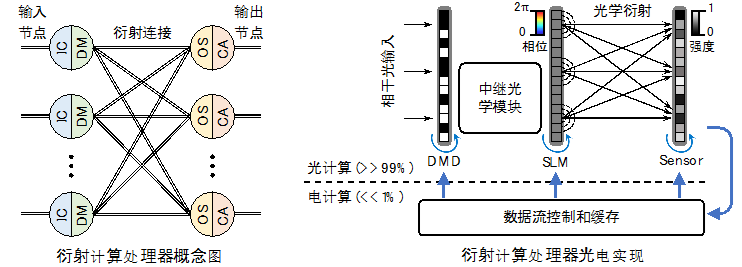
Fig. 1. The fundamental principle of optoelectronic AI diffractive processing unit (DPU)
In order to tackle the above challenges, a research group led by Professor Qionghai Dai from the School of Information Science and Technology at Tsinghua University proposed and built an optoelectronic reconfigurable diffractive processing unit (DPU), which can be effectively reconfigured to implement multiple different types of novel optoelectronic neural networks containing millions of neurons. With the proposed adaptive training algorithm, high-performance visual classification tasks were achieved. Besides, the optoelectronic intelligent computing advantage has been verified.
The architecture of DPU uses the physical phenomenon of optical diffraction for generating large-scale optical interconnections, which facilitates the construction of high-complexity optical neural networks (Fig. 1). In addition, the proposed optical processor makes full use of the wave-particle duality of light: the adjustment of neural network weights is achieved by controlling the wavefront profile of lightwave propagation; the photoelectric effect realizes the artificial neuron functionality. The optical computing part of the DPU performs almost all computing operations. By using high-throughput programmable optoelectronic devices and combining the flexible characteristics of electronic computing, it achieves high-speed data control and large-scale network structure and parameter programming. "In this work, we defined the optoelectronic diffractive artificial neuron, which achieves linear weighted summation of the diffracted light field as well as the nonlinear activation response. This is the basis for constructing the complex optoelectronic deep learning system." Xing Lin said, who is now a distinguished research fellow at Tsinghua University.
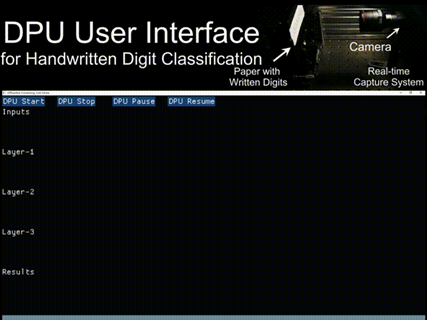
Fig. 2. Handwritten digit classification with DPU
Reconstructing the DPU through software programming achieves multiple types of deep neural network architectures, including feedforward and recurrent neural networks. Each neural network contains millions of neurons and has a high model complexity. With the proposed adaptive training method for correcting the accumulation of system errors, the experimental computing performance of neural networks was optimized. The adaptive training method implements real-time adjustment of calculation parameters by measuring the internal state of the neural network, which does not rely on the network structure and can be universally adapted to the existing optoelectronic intelligent computing systems.
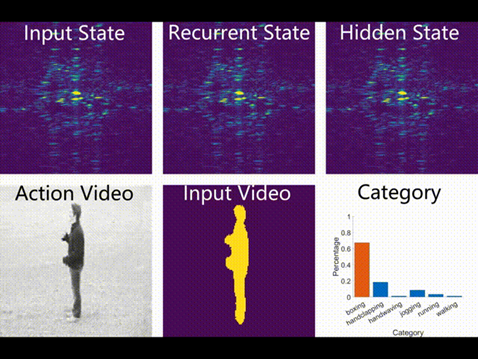
Fig. 3. Human action recognition with DPU
The constructed system has been applied to the image and video recognition tasks, and its performance was verified on the standard deep learning databases, including handwritten digit database (MNIST, see Fig. 2), fashion product database (Fashion-MNIST), and human action database (Weizmann and KTH, see Fig. 3). The performance of the proposed optoelectronic intelligent computing model surpasses the LeNet-4 electronic neural network model for the first time. The system can also achieve high-speed, high-precision human action recognition. Running the same neural networks, the optoelectronic computing system is 8 times faster than the Tesla V100 graphics processing unit (GPU), the system energy efficiency is improved by more than an order of magnitude, and the core computing energy efficiency is improved by four orders of magnitude.
Professor Qionghai Dai pointed out, “The potential of current optoelectronic intelligent computing technology has yet to be explored in depth. The use of metamaterials to build on-chip phased arrays in the future can greatly improve the computing performance of the processor, while achieving the goal of optoelectronic computers requires interdisciplinary research." The future of optoelectronic computing is coming. The progress of brain-inspired optoelectronic chips will greatly promote the development of artificial intelligence. The work will lay the foundation for the real-time intelligent processing of large-scale data, high-speed and low-power intelligent unmanned systems, and from billion pixels to tens of billions pixel light field imaging.
This work was published in the journal Nature Photonics, with Ph.D. student Tiankuang Zhou as the first author of the paper, Distinguished Research Fellow Xing Lin (Tsinghua Institute of Brain and Cognitive Sciences), Associate Professor Lu Fang (Department of Electronics, Tsinghua University), and Professor Dai Qionghai (Beijing National Research Center for Information Science and Technology) as the corresponding authors. The work was supported by the Ministry of Science and Technology of China and the National Natural Science Foundation of China.
[1] Zhou, Tiankuang, Xing Lin, Jiamin Wu, Yitong Chen, Hao Xie, Yipeng Li, Jingtao Fan, Huaqiang Wu, Lu Fang, and Qionghai Dai. "Large-scale neuromorphic optoelectronic computing with a reconfigurable diffractive processing unit." Nature Photonics 15, no. 5 (2021): 367-373.